Understanding the AI-Data Privacy Conundrum
AI’s ability to analyze vast amounts of data brings unparalleled insights and advancements. However, this capability also raises significant data privacy concerns. From unauthorized data access to the misuse of personal information, the challenges are multifaceted. Balancing innovation with privacy protection is the crux of this conundrum.
Emerging Trends in AI and Data Privacy
Several key trends are emerging at the intersection of AI and data privacy. These trends are driven by the need to enhance data security, comply with regulatory standards, and maintain public trust.
1. Privacy-Preserving Machine Learning (PPML)
Privacy-preserving machine learning (PPML) is a set of techniques that allow AI models to be trained on sensitive data without exposing the data itself. Methods such as federated learning, differential privacy, and homomorphic encryption are at the forefront of PPML.
Technique | Description | Example |
---|---|---|
Federated Learning | Training models across multiple devices without centralizing data. | Google AI |
Differential Privacy | Ensuring that the output of AI models does not compromise individual data points. | Harvard Privacy Tools Project |
Homomorphic Encryption | Enabling computations on encrypted data without decrypting it. | Microsoft Research |
2. Regulatory Compliance and Ethical AI
As AI technologies advance, regulatory bodies worldwide are establishing stricter data privacy laws. The General Data Protection Regulation (GDPR) in Europe and the California Consumer Privacy Act (CCPA) in the United States are notable examples. These regulations enforce stringent data protection measures and emphasize the ethical use of AI.
3. AI-Driven Data Anonymization
Data anonymization techniques are evolving with AI’s help, making it possible to derive insights from data while protecting individual privacy. Advanced anonymization algorithms can effectively remove personally identifiable information (PII) without compromising data utility.
Cutting-Edge Technologies Enhancing Data Privacy
Technological advancements are pivotal in enhancing data privacy. Here are some key technologies making waves in this space:
1. Blockchain Technology
Blockchain’s decentralized nature offers a robust framework for secure data transactions. By providing transparency and immutability, blockchain can ensure data integrity and prevent unauthorized access. Projects like Enigma and Oasis Labs are leveraging blockchain to enhance data privacy.
2. Zero-Knowledge Proofs (ZKPs)
Zero-knowledge proofs (ZKPs) are cryptographic methods that enable one party to prove to another that they know a value without revealing the value itself. ZKPs are being utilized to enhance data privacy in various applications, from secure voting systems to confidential transactions.
3. Secure Multi-Party Computation (SMPC)
Secure multi-party computation (SMPC) allows multiple parties to collaboratively compute a function over their inputs while keeping those inputs private. This technique is gaining traction in fields like finance, healthcare, and national security where data privacy is paramount.
4. Quantum-Safe Encryption
As quantum computing progresses, traditional encryption methods may become vulnerable. Quantum-safe encryption algorithms are being developed to protect data against potential quantum attacks. Organizations like ID Quantique are at the forefront of this innovation.
Challenges and Future Directions
While advancements in AI and data privacy are promising, several challenges remain. These include the scalability of privacy-preserving techniques, the need for robust regulatory frameworks, and the ethical considerations surrounding AI use. Addressing these challenges will be crucial for the future of data privacy.
1. Scalability Issues
Scalability is a significant concern in implementing privacy-preserving techniques. Techniques like homomorphic encryption and SMPC are computationally intensive, which can hinder their widespread adoption. Research and development efforts are focused on making these techniques more efficient and scalable.
2. Regulatory Harmonization
With varying data privacy regulations across different regions, achieving regulatory harmonization is challenging. International cooperation and the establishment of global data privacy standards are necessary to create a cohesive framework for data protection.
3. Ethical AI Development
The ethical implications of AI use are a critical consideration. Ensuring that AI systems are transparent, accountable, and free from biases is essential for maintaining public trust. Ethical guidelines and standards for AI development must be established and rigorously enforced.
Conclusion
The future of data privacy in the age of AI is a dynamic and evolving landscape. Emerging trends and technologies are paving the way for more secure and privacy-conscious AI applications. By addressing the challenges and embracing innovative solutions, we can harness the power of AI while safeguarding personal data and maintaining public trust.
Pros of AI in Data Privacy
1. Enhanced Data Security
AI-driven security systems can detect and respond to data breaches more quickly and accurately than traditional methods. Machine learning algorithms analyze patterns and anomalies in real-time, enabling proactive measures to prevent unauthorized access.
Benefit | Description | Example |
---|---|---|
Anomaly Detection | Identifying unusual patterns that may indicate security threats. | IBM Security AI |
Automated Responses | Immediate actions taken by AI systems to mitigate data breaches. | CrowdStrike |
2. Efficient Data Management
AI enhances data management by automating processes such as data classification, indexing, and retrieval. This not only improves efficiency but also reduces the risk of human error in handling sensitive information.
For instance, AI-powered data discovery tools can classify and tag data based on its sensitivity, ensuring that personal information is adequately protected. These tools streamline compliance with data protection regulations by ensuring that all data is properly categorized and secured.
3. Privacy-Preserving Data Analysis
AI enables privacy-preserving data analysis techniques such as federated learning and differential privacy. These methods allow for the extraction of valuable insights from data without compromising individual privacy.
Federated learning, for example, trains machine learning models on decentralized data sources, ensuring that raw data remains local and private. Differential privacy adds noise to datasets, making it difficult to identify individual data points while still allowing for accurate analysis.
4. Compliance Automation
AI tools can automate compliance with data protection regulations such as GDPR and CCPA. These tools help organizations monitor and manage data privacy practices, ensuring adherence to legal requirements and reducing the risk of non-compliance penalties.
Automated compliance systems can track data usage, manage consent, and generate audit trails, making it easier for organizations to demonstrate their commitment to data privacy. This not only enhances regulatory compliance but also builds trust with customers.
Cons of AI in Data Privacy
1. Data Privacy Risks
While AI can enhance data security, it also introduces new privacy risks. AI systems require large amounts of data for training and operation, which can lead to data breaches if not properly managed. Additionally, AI algorithms can inadvertently reveal sensitive information if not designed with privacy in mind.
For example, machine learning models trained on biased or unprotected data can produce outputs that compromise individual privacy. Ensuring that AI systems are developed and tested with robust privacy safeguards is crucial to mitigating these risks.
2. Ethical Concerns
The use of AI in data privacy raises ethical concerns, particularly regarding transparency, accountability, and bias. AI algorithms can perpetuate existing biases in data, leading to unfair treatment of certain individuals or groups.
Moreover, the lack of transparency in AI decision-making processes can make it difficult to understand how privacy decisions are made. Ensuring that AI systems are transparent, accountable, and free from bias is essential to address these ethical challenges.
3. Complexity and Cost
Implementing AI-driven data privacy solutions can be complex and costly. Developing, testing, and maintaining AI systems require significant investment in technology and expertise. Small and medium-sized enterprises (SMEs) may find it challenging to adopt AI-based privacy solutions due to resource constraints.
Additionally, the integration of AI into existing data privacy frameworks can be a complex process, requiring careful planning and execution to ensure compatibility and effectiveness.
4. Regulatory Challenges
The rapid evolution of AI technologies often outpaces regulatory frameworks, leading to challenges in ensuring compliance with data protection laws. Regulatory bodies may struggle to keep up with the pace of AI development, resulting in gaps and inconsistencies in data privacy regulations.
Organizations must navigate these regulatory challenges while ensuring that their AI systems adhere to current and future data privacy requirements. This necessitates a proactive approach to regulatory compliance and continuous monitoring of the evolving legal landscape.
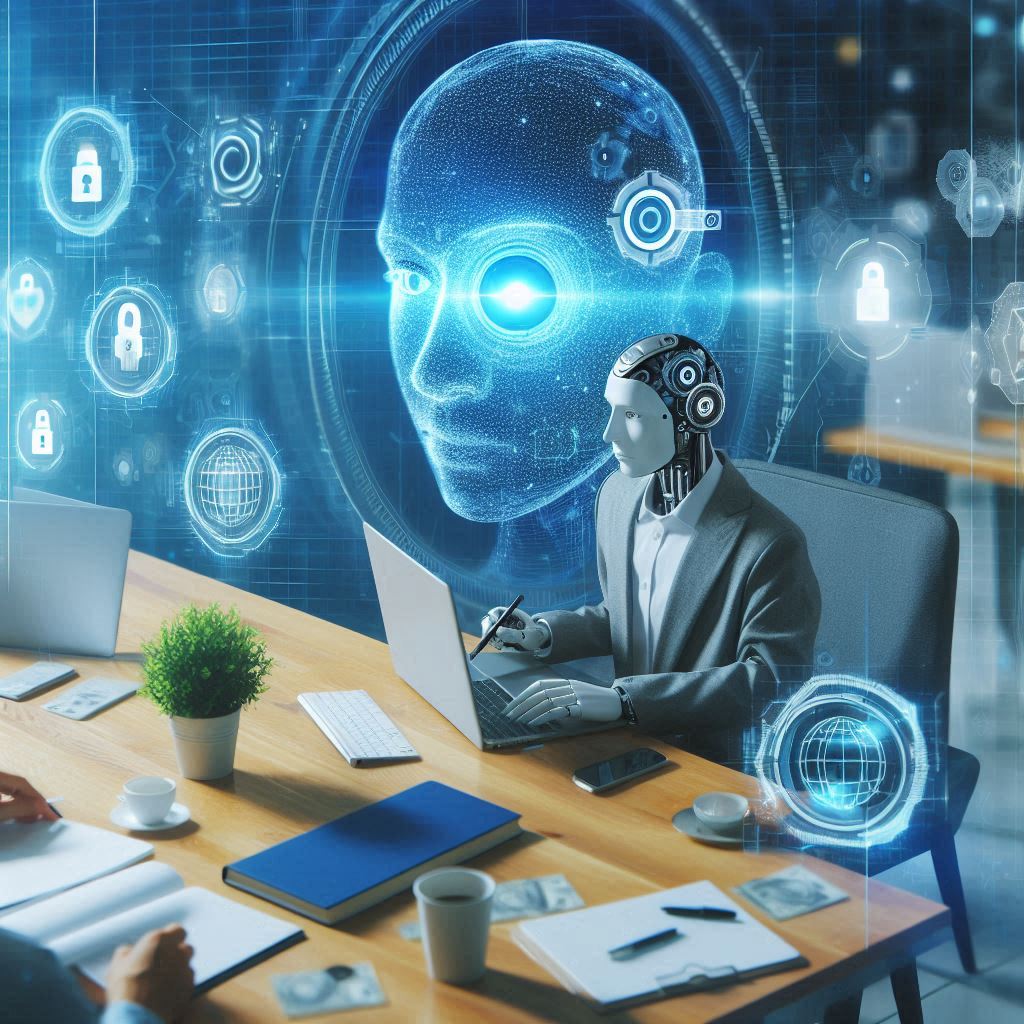
Conclusion
The integration of AI into data privacy practices offers significant benefits, including enhanced data security, efficient data management, privacy-preserving analysis, and automated compliance. However, it also introduces new risks and challenges, such as data privacy risks, ethical concerns, complexity and cost, and regulatory challenges.
Balancing the pros and cons of AI in data privacy requires a careful and informed approach. By addressing the potential downsides and leveraging the advantages, organizations can harness the power of AI to protect personal data and maintain public trust in the digital age.
1. What is the role of AI in data privacy?
AI plays a crucial role in enhancing data privacy by providing advanced tools and techniques for data protection. AI can help identify and mitigate security threats, automate compliance with data protection regulations, and enable privacy-preserving data analysis methods. However, AI also requires access to large datasets, which can pose privacy risks if not managed properly.
2. How does AI enhance data security?
AI enhances data security through several mechanisms:
- Anomaly Detection: AI systems can identify unusual patterns and behaviors that may indicate a security breach.
- Automated Responses: AI can automatically respond to detected threats, mitigating potential damage in real-time.
- Predictive Analysis: AI can predict and prevent future security incidents by analyzing historical data.
3. What are privacy-preserving AI techniques?
Privacy-preserving AI techniques allow AI models to be trained and operated without compromising the privacy of the data involved. Key techniques include:
- Federated Learning: This method involves training AI models across decentralized devices or servers holding local data samples, without sharing the data itself.
- Differential Privacy: Adding noise to the data or to the model’s outputs to protect individual data points while still allowing for useful analysis.
- Homomorphic Encryption: Enabling computations on encrypted data, ensuring that sensitive data remains encrypted throughout the process.
4. How does AI help with regulatory compliance?
AI helps with regulatory compliance by automating the monitoring and management of data privacy practices. AI tools can:
- Track data usage and ensure it adheres to privacy policies and regulations.
- Manage user consent and preferences.
- Generate audit trails and compliance reports.
- Detect and respond to compliance breaches in real-time.
5. What are the risks of using AI in data privacy?
While AI can significantly enhance data privacy, it also introduces several risks:
- Data Privacy Risks: AI systems require large datasets, which can lead to data breaches if not managed properly.
- Bias and Discrimination: AI models can perpetuate existing biases in data, leading to unfair treatment of individuals or groups.
- Lack of Transparency: AI decision-making processes can be opaque, making it difficult to understand how privacy decisions are made.
- Regulatory Compliance: Rapid advancements in AI can outpace regulatory frameworks, making compliance challenging.
6. How can organizations mitigate the risks associated with AI in data privacy?
Organizations can mitigate the risks associated with AI in data privacy by adopting several best practices:
- Implement Robust Data Management Practices: Ensure that data is collected, stored, and processed securely, with appropriate access controls.
- Conduct Regular Audits: Regularly audit AI systems and data privacy practices to identify and address vulnerabilities.
- Adopt Privacy-Preserving Techniques: Use techniques like federated learning, differential privacy, and homomorphic encryption to protect sensitive data.
- Ensure Transparency and Accountability: Make AI decision-making processes transparent and establish clear accountability for data privacy practices.
- Stay Informed on Regulatory Changes: Keep abreast of changes in data privacy regulations and ensure compliance with current and future requirements.
7. What are the ethical considerations in using AI for data privacy?
Ethical considerations in using AI for data privacy include ensuring that AI systems are transparent, accountable, and free from biases. Organizations must consider the potential impact of AI on individuals’ privacy rights and strive to develop AI systems that respect and protect those rights. Ethical guidelines and standards should be established and rigorously enforced to maintain public trust in AI technologies.
8. How can AI protect sensitive data in healthcare?
In healthcare, AI can protect sensitive data through advanced data encryption, anonymization, and secure data sharing methods. Privacy-preserving techniques like federated learning can enable collaborative research and medical insights without exposing patient data. AI-driven systems can also monitor and detect unauthorized access to patient records, ensuring compliance with healthcare privacy regulations like HIPAA.
9. What is the future of AI and data privacy?
The future of AI and data privacy will likely involve continued advancements in privacy-preserving technologies, stricter regulatory frameworks, and increased public awareness of data privacy issues. AI will play a pivotal role in developing more sophisticated and secure data protection methods, ensuring that privacy is maintained even as AI capabilities expand. Collaboration between tech companies, regulatory bodies, and privacy advocates will be crucial in shaping a future where AI and data privacy coexist harmoniously.
10. How can individuals protect their data privacy in the age of AI?
Individuals can protect their data privacy in the age of AI by adopting several practices:
- Be Informed: Stay informed about how your data is collected, used, and shared by AI systems.
- Use Privacy Tools: Utilize privacy-enhancing tools and settings provided by apps and online services.
- Limit Data Sharing: Be cautious about sharing personal information online and only provide necessary data.
- Manage Consent: Regularly review and manage consent preferences for data collection and usage.
- Monitor Accounts: Keep an eye on your online accounts for any suspicious activity and use strong, unique passwords.
Conclusion
The integration of AI into data privacy practices presents both opportunities and challenges. By understanding the role of
1. What is the role of AI in data privacy?
AI plays a crucial role in enhancing data privacy by providing advanced tools and techniques for data protection. AI can help identify and mitigate security threats, automate compliance with data protection regulations, and enable privacy-preserving data analysis methods. However, AI also requires access to large datasets, which can pose privacy risks if not managed properly.
2. How does AI enhance data security?
AI enhances data security through several mechanisms:
- Anomaly Detection: AI systems can identify unusual patterns and behaviors that may indicate a security breach.
- Automated Responses: AI can automatically respond to detected threats, mitigating potential damage in real-time.
- Predictive Analysis: AI can predict and prevent future security incidents by analyzing historical data.
3. What are privacy-preserving AI techniques?
Privacy-preserving AI techniques allow AI models to be trained and operated without compromising the privacy of the data involved. Key techniques include:
- Federated Learning: This method involves training AI models across decentralized devices or servers holding local data samples, without sharing the data itself.
- Differential Privacy: Adding noise to the data or to the model’s outputs to protect individual data points while still allowing for useful analysis.
- Homomorphic Encryption: Enabling computations on encrypted data, ensuring that sensitive data remains encrypted throughout the process.
4. How does AI help with regulatory compliance?
AI helps with regulatory compliance by automating the monitoring and management of data privacy practices. AI tools can:
- Track data usage and ensure it adheres to privacy policies and regulations.
- Manage user consent and preferences.
- Generate audit trails and compliance reports.
- Detect and respond to compliance breaches in real-time.
5. What are the risks of using AI in data privacy?
While AI can significantly enhance data privacy, it also introduces several risks:
- Data Privacy Risks: AI systems require large datasets, which can lead to data breaches if not managed properly.
- Bias and Discrimination: AI models can perpetuate existing biases in data, leading to unfair treatment of individuals or groups.
- Lack of Transparency: AI decision-making processes can be opaque, making it difficult to understand how privacy decisions are made.
- Regulatory Compliance: Rapid advancements in AI can outpace regulatory frameworks, making compliance challenging.
6. How can organizations mitigate the risks associated with AI in data privacy?
Organizations can mitigate the risks associated with AI in data privacy by adopting several best practices:
- Implement Robust Data Management Practices: Ensure that data is collected, stored, and processed securely, with appropriate access controls.
- Conduct Regular Audits: Regularly audit AI systems and data privacy practices to identify and address vulnerabilities.
- Adopt Privacy-Preserving Techniques: Use techniques like federated learning, differential privacy, and homomorphic encryption to protect sensitive data.
- Ensure Transparency and Accountability: Make AI decision-making processes transparent and establish clear accountability for data privacy practices.
- Stay Informed on Regulatory Changes: Keep abreast of changes in data privacy regulations and ensure compliance with current and future requirements.
7. What are the ethical considerations in using AI for data privacy?
Ethical considerations in using AI for data privacy include ensuring that AI systems are transparent, accountable, and free from biases. Organizations must consider the potential impact of AI on individuals’ privacy rights and strive to develop AI systems that respect and protect those rights. Ethical guidelines and standards should be established and rigorously enforced to maintain public trust in AI technologies.
8. How can AI protect sensitive data in healthcare?
In healthcare, AI can protect sensitive data through advanced data encryption, anonymization, and secure data sharing methods. Privacy-preserving techniques like federated learning can enable collaborative research and medical insights without exposing patient data. AI-driven systems can also monitor and detect unauthorized access to patient records, ensuring compliance with healthcare privacy regulations like HIPAA.
9. What is the future of AI and data privacy?
The future of AI and data privacy will likely involve continued advancements in privacy-preserving technologies, stricter regulatory frameworks, and increased public awareness of data privacy issues. AI will play a pivotal role in developing more sophisticated and secure data protection methods, ensuring that privacy is maintained even as AI capabilities expand. Collaboration between tech companies, regulatory bodies, and privacy advocates will be crucial in shaping a future where AI and data privacy coexist harmoniously.
10. How can individuals protect their data privacy in the age of AI?
Individuals can protect their data privacy in the age of AI by adopting several practices:
- Be Informed: Stay informed about how your data is collected, used, and shared by AI systems.
- Use Privacy Tools: Utilize privacy-enhancing tools and settings provided by apps and online services.
- Limit Data Sharing: Be cautious about sharing personal information online and only provide necessary data.
- Manage Consent: Regularly review and manage consent preferences for data collection and usage.
- Monitor Accounts: Keep an eye on your online accounts for any suspicious activity and use strong, unique passwords.
Conclusion
The integration of AI into data privacy practices presents both opportunities and challenges. By understanding the role of
Disclaimer
The information provided in this article is for informational purposes only. While every effort has been made to ensure the accuracy and reliability of the information presented, AI and data privacy are rapidly evolving fields, and new developments may occur that could impact the accuracy of the information provided. Readers are advised to conduct their own research and consult with qualified professionals regarding specific issues related to AI, data privacy, and legal compliance.
Furthermore, the views and opinions expressed in this article are those of the author and do not necessarily reflect the official policy or position of any organization or entity mentioned. The inclusion of third-party links, references, or examples does not constitute an endorsement or recommendation.
Caution
While AI offers significant potential benefits in terms of enhancing data privacy, it also introduces several risks and challenges that must be carefully considered:
- Data Security Risks: AI systems require access to large datasets for training and operation, which can pose security risks if not adequately protected. Organizations must implement robust data security measures to prevent unauthorized access and data breaches.
- Privacy Concerns: The use of AI in data privacy can raise concerns about the protection of individuals’ privacy rights. AI algorithms may inadvertently reveal sensitive information or perpetuate biases in data, leading to potential ethical and legal implications.
- Ethical Considerations: AI decision-making processes must be transparent, accountable, and free from biases to ensure fairness and avoid unintended consequences. Organizations should adhere to ethical guidelines and standards to mitigate the risks of AI-driven decision-making.
- Regulatory Compliance: Rapid advancements in AI technology often outpace regulatory frameworks, creating challenges in ensuring compliance with data protection laws and regulations. Organizations must stay informed about regulatory changes and adapt their AI systems accordingly to avoid legal liabilities.
- Complexity and Implementation Challenges: Implementing AI-driven data privacy solutions can be complex and costly, requiring expertise in both AI technologies and legal compliance. Small and medium-sized enterprises (SMEs) may face resource constraints and technical challenges in adopting AI-based privacy solutions.
Conclusion
In conclusion, while AI holds immense promise for advancing data privacy practices, it is essential to approach its implementation with caution and diligence. By understanding the potential risks, addressing privacy concerns, and ensuring compliance with regulatory requirements, organizations can harness the full potential of AI while safeguarding individuals’ privacy rights. Continued research, collaboration, and transparency are crucial in navigating the evolving landscape of AI and data privacy.