The Intersection of AI and Ethics: Addressing Bias in Machine Learning
Artificial Intelligence (AI) has revolutionized many industries, but with its rise, ethical concerns have also emerged, particularly regarding bias in machine learning. This article delves into the complexities of AI ethics, showcasing success stories, best practices, and strategies to mitigate bias in machine learning systems.
Understanding Bias in Machine Learning
Bias in machine learning refers to systematic errors that result in unfair outcomes, often disadvantaging certain groups. These biases can stem from various sources, including training data, algorithm design, and human oversight.
Types of Bias
Type of Bias | Description |
---|---|
Historical Bias | Bias resulting from existing prejudices in historical data. |
Sampling Bias | Bias occurring when the training data is not representative of the overall population. |
Measurement Bias | Bias introduced by inaccurate measurements or data collection methods. |
Algorithmic Bias | Bias arising from the design and implementation of machine learning algorithms. |
Success Stories and Exemplary Cases
Numerous organizations have emerged as leaders in addressing AI bias, showcasing how ethical AI practices can lead to successful and fair outcomes. Here are some notable examples:
IBM has been at the forefront of promoting ethical AI. Their Watson AI Ethics initiative focuses on creating transparent, fair, and accountable AI systems. They have developed tools to detect and mitigate bias in their AI models.
Google has also made significant strides in this area. Their AI Ethics and Fairness team works on ensuring their AI technologies are fair and unbiased. Google’s What-If Tool allows developers to visualize the effects of different biases and make adjustments accordingly.
Microsoft has implemented the Responsible AI initiative, which emphasizes ethical AI development. Their Fairlearn toolkit helps developers assess and mitigate fairness issues in machine learning models.
Strategies to Mitigate Bias in Machine Learning
Addressing bias in machine learning requires a multifaceted approach. Here are some effective strategies:
1. Diverse and Representative Data
Ensuring that training data is diverse and representative of the target population is crucial. This helps in minimizing sampling bias and making the AI system more equitable.
2. Bias Detection and Mitigation Tools
Using tools like IBM’s AI Fairness 360, Google’s What-If Tool, and Microsoft’s Fairlearn can help identify and correct biases in machine learning models.
3. Transparent and Explainable AI
Developing transparent and explainable AI models allows stakeholders to understand how decisions are made, which can help in identifying and addressing biases.
4. Ethical Guidelines and Policies
Establishing clear ethical guidelines and policies for AI development and deployment ensures that all stakeholders are committed to fairness and accountability.
Future Trends and Considerations
As AI continues to evolve, addressing ethical concerns will remain paramount. Future trends in AI ethics include:
1. Enhanced Regulatory Frameworks
Governments and organizations are expected to develop stricter regulations and frameworks to ensure ethical AI practices.
2. Increased Focus on Fairness and Transparency
There will be a greater emphasis on creating AI systems that are not only fair but also transparent, allowing for better oversight and accountability.
3. Collaboration Across Sectors
Collaboration between academia, industry, and government will be crucial in addressing ethical challenges and developing best practices for AI.
4. Continuous Monitoring and Evaluation
Ongoing monitoring and evaluation of AI systems will be necessary to identify and mitigate any emerging biases or ethical issues.
In conclusion, the intersection of AI and ethics is a critical area that requires continuous attention and action. By implementing best practices and leveraging technological advancements, we can create AI systems that are fair, transparent, and beneficial for all.
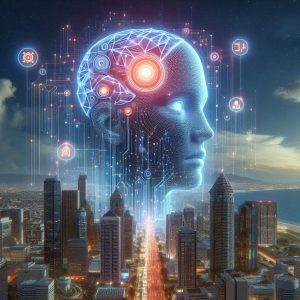
Pros and Cons of AI in Addressing Bias in Machine Learning
The intersection of AI and ethics is complex, particularly when it comes to addressing bias in machine learning. Understanding the pros and cons of AI in this context is essential for developing fair and effective AI systems. This section outlines the advantages and challenges associated with AI and bias in machine learning.
Pros
1. Improved Decision-Making
AI systems can analyze vast amounts of data to make informed decisions, reducing human error and subjectivity. This leads to more consistent and objective outcomes.
2. Enhanced Fairness
When designed and implemented correctly, AI can help mitigate existing biases in decision-making processes. Tools like IBM’s Watson AI Ethics and Google’s What-If Tool enable developers to identify and correct biases.
3. Scalability
AI systems can be scaled to handle large datasets and complex tasks that would be infeasible for humans, ensuring broader and more comprehensive analyses.
4. Continuous Learning and Improvement
Machine learning models can continuously learn from new data, improving their accuracy and fairness over time. This adaptive nature helps in addressing biases that may emerge as societal norms evolve.
5. Enhanced Transparency and Accountability
Developing transparent and explainable AI models allows stakeholders to understand the decision-making process, fostering accountability and trust. Microsoft’s Fairlearn toolkit is an example of such efforts.
Cons
1. Data Quality and Representation Issues
AI systems are heavily dependent on the quality and representativeness of the data they are trained on. Poor quality or biased data can lead to flawed AI models, perpetuating or even exacerbating existing biases.
2. Algorithmic Bias
Even with high-quality data, biases can still arise from the design and implementation of algorithms. These biases can be difficult to detect and correct, potentially leading to unfair outcomes.
3. Lack of Transparency
Many AI models, particularly deep learning systems, operate as “black boxes,” making it challenging to understand how decisions are made. This lack of transparency can hinder efforts to identify and address biases.
4. Ethical and Legal Challenges
The ethical and legal landscape surrounding AI is still evolving. There are significant challenges in establishing clear guidelines and regulations to ensure that AI systems are used ethically and responsibly.
5. Risk of Over-Reliance on AI
Over-reliance on AI systems can lead to a loss of human oversight and critical thinking. It is essential to maintain a balance between AI-driven decision-making and human judgment to avoid potential pitfalls.
Conclusion
While AI holds significant potential to address bias in machine learning, it is not without its challenges. By understanding the pros and cons, stakeholders can develop more ethical and effective AI systems. Continuous efforts in improving data quality, algorithmic transparency, and regulatory frameworks are essential to harness the benefits of AI while mitigating its risks.
Frequently Asked Questions (FAQs) on AI and Ethics: Addressing Bias in Machine Learning
In the rapidly evolving field of AI and machine learning, addressing bias is a critical concern. This FAQ section aims to provide clear and concise answers to common questions about AI ethics and bias in machine learning.
1. What is bias in machine learning?
Bias in machine learning refers to systematic errors that result in unfair outcomes, often disadvantaging certain groups. These biases can arise from various sources, including training data, algorithm design, and human oversight.
2. What are the different types of bias in machine learning?
There are several types of bias in machine learning:
- Historical Bias: Bias that stems from existing prejudices in historical data.
- Sampling Bias: Bias that occurs when the training data is not representative of the overall population.
- Measurement Bias: Bias introduced by inaccurate measurements or data collection methods.
- Algorithmic Bias: Bias arising from the design and implementation of machine learning algorithms.
3. How can AI help mitigate bias in machine learning?
AI can help mitigate bias in several ways:
- Diverse and Representative Data: Using diverse and representative training data can minimize sampling bias and ensure equitable AI systems.
- Bias Detection and Mitigation Tools: Tools like IBM’s Watson AI Ethics, Google’s What-If Tool, and Microsoft’s Fairlearn toolkit can identify and correct biases in machine learning models.
- Transparent and Explainable AI: Developing transparent and explainable AI models allows stakeholders to understand decision-making processes and identify biases.
4. What are the challenges of addressing bias in machine learning?
Some challenges of addressing bias in machine learning include:
- Data Quality and Representation: Ensuring high-quality and representative data can be difficult, but it is essential to minimize bias.
- Algorithmic Bias: Biases can still arise from the design and implementation of algorithms, even with good data.
- Lack of Transparency: Many AI models operate as “black boxes,” making it hard to understand how decisions are made and identify biases.
- Ethical and Legal Challenges: The evolving ethical and legal landscape surrounding AI requires clear guidelines and regulations to ensure responsible use.
- Over-Reliance on AI: There is a risk of losing human oversight and critical thinking if we over-rely on AI systems.
5. What are some examples of organizations addressing AI bias?
Several organizations are leading the way in addressing AI bias:
- IBM: Their Watson AI Ethics initiative focuses on creating transparent, fair, and accountable AI systems.
- Google: Their AI Ethics and Fairness team works on ensuring their AI technologies are fair and unbiased. Their What-If Tool allows developers to visualize and mitigate biases.
- Microsoft: Their Responsible AI initiative emphasizes ethical AI development, with tools like Fairlearn helping developers assess and mitigate fairness issues.
6. How can developers ensure their AI systems are fair and unbiased?
Developers can ensure their AI systems are fair and unbiased by:
- Using Diverse and Representative Data: Ensuring that the training data represents the target population helps minimize bias.
- Implementing Bias Detection Tools: Leveraging tools like IBM’s AI Fairness 360, Google’s What-If Tool, and Microsoft’s Fairlearn can help identify and correct biases.
- Developing Transparent Models: Creating transparent and explainable AI models allows for better understanding and identification of biases.
- Establishing Ethical Guidelines: Following clear ethical guidelines and policies ensures a commitment to fairness and accountability.
7. What are future trends in AI ethics and bias mitigation?
Future trends in AI ethics and bias mitigation include:
- Enhanced Regulatory Frameworks: Stricter regulations and frameworks are expected to ensure ethical AI practices.
- Increased Focus on Fairness and Transparency: There will be a greater emphasis on creating fair and transparent AI systems.
- Collaboration Across Sectors: Collaboration between academia, industry, and government will be crucial in addressing ethical challenges and developing best practices.
- Continuous Monitoring and Evaluation: Ongoing monitoring and evaluation of AI systems will be necessary to identify and mitigate emerging biases and ethical issues.
8. How can organizations implement ethical AI practices?
Organizations can implement ethical AI practices by:
- Establishing Clear Ethical Guidelines: Developing and adhering to ethical guidelines for AI development and deployment.
- Training and Education: Providing training and education to stakeholders on the importance of AI ethics and how to implement best practices.
- Using Ethical AI Tools: Leveraging tools designed to detect and mitigate bias, such as IBM’s Watson AI Ethics, Google’s What-If Tool, and Microsoft’s Fairlearn.
- Promoting Transparency and Accountability: Ensuring that AI systems
Disclaimer and Caution on AI and Ethics: Addressing Bias in Machine Learning
The content provided in this article is for informational purposes only and does not constitute professional advice, legal guidance, or a comprehensive treatment of the subject matter. The topic of AI and ethics, particularly the issue of bias in machine learning, is highly complex and continuously evolving. Readers are encouraged to seek professional consultation and conduct further research to understand fully the implications and best practices in this area.
Understanding Limitations
The field of AI and machine learning is rapidly advancing, and while significant strides have been made in addressing bias, the solutions and strategies mentioned in this article may not be exhaustive or universally applicable. The effectiveness of these strategies can vary based on the specific context, type of data, and particular application of AI.
Potential Risks and Uncertainties
It is important to acknowledge that despite best efforts, AI systems may still exhibit biases. These biases can have significant ethical, social, and legal implications. Organizations should be aware of the potential risks and uncertainties involved in developing and deploying AI technologies. Continuous monitoring and evaluation are essential to identify and mitigate any unintended consequences.
No Guarantee of Bias Elimination
The strategies and tools discussed in this article are designed to mitigate bias but cannot guarantee the complete elimination of bias. Bias in AI can stem from various sources, including data quality, algorithmic design, and human oversight. As such, ongoing vigilance and adaptation of strategies are necessary to address emerging biases.
Legal and Ethical Responsibilities
Organizations and individuals developing and deploying AI systems must adhere to relevant legal and ethical standards. This includes compliance with data protection laws, anti-discrimination laws, and industry-specific regulations. Ethical AI practices should be integrated into all stages of AI development and deployment, from data collection to model implementation and beyond.
Collaboration and Stakeholder Engagement
Addressing bias in AI requires collaboration across various stakeholders, including developers, researchers, policymakers, and affected communities. Engaging diverse perspectives and expertise can help identify potential biases and develop more robust and inclusive AI systems. Transparent communication and ethical guidelines are crucial in fostering trust and accountability.
Dynamic Nature of AI Ethics
The ethical considerations of AI are not static and will continue to evolve as technology advances and societal norms change. Organizations must remain proactive in updating their ethical guidelines and practices in response to new developments and challenges in the field. Staying informed about the latest research, regulatory changes, and industry best practices is essential.
Educational and Training Initiatives
Organizations should invest in ongoing education and training for their teams to ensure a thorough understanding of AI ethics and bias. This includes awareness of the potential for bias, methods for mitigating it, and the importance of maintaining transparency and accountability. Regular training can help embed ethical considerations into the organizational culture and decision-making processes.
Use of Bias Detection and Mitigation Tools
While tools like IBM’s Watson AI Ethics, Google’s What-If Tool, and Microsoft’s Fairlearn toolkit are valuable in identifying and mitigating bias, they should not be viewed as a panacea. These tools are part of a broader strategy that includes careful data management, transparent algorithm development, and comprehensive testing and validation.
Ethical Considerations in Data Collection
The data used to train AI models plays a critical role in determining the fairness of the outcomes. Ethical data collection practices, including obtaining informed consent, ensuring data diversity, and respecting privacy, are essential. Organizations should implement rigorous data governance frameworks to maintain the integrity and ethical use of data.
Stakeholder Responsibility
All stakeholders involved in AI development and deployment share the responsibility of ensuring ethical practices. This includes developers, data scientists, business leaders, and policymakers. Each group must understand their role in mitigating bias and promoting fairness in AI systems.
Conclusion
While AI holds significant potential to enhance decision-making and fairness, addressing bias is a multifaceted challenge that requires continuous effort and vigilance. This disclaimer and caution aim to highlight the complexities and responsibilities involved in developing ethical AI systems. By acknowledging the limitations and potential risks, and by committing to ongoing education, collaboration, and ethical practices, organizations can work towards creating more fair and accountable AI technologies.
[…] of AI in storytelling requires a balanced approach that fosters innovation while upholding ethical standards and creative integrity. Establishing clear ethical guidelines and regulatory frameworks can […]