Tackling AI Bias Strategies for Ethical Development and Deployment
Artificial Intelligence (AI) has become an integral part of various sectors, from healthcare and finance to education and entertainment. However, the growing reliance on AI has also brought to light significant concerns about bias in AI systems. Addressing AI bias is crucial for ensuring ethical development and deployment. This article explores strategies to tackle AI bias, featuring success stories, practical approaches, and external links to reputable sources for further reading.
Understanding AI Bias
AI bias occurs when an AI system produces results that are systematically prejudiced due to erroneous assumptions in the machine learning process. These biases can emerge from training data, algorithms, or the deployment environment, leading to unfair treatment of certain groups. For a deeper understanding of AI bias, refer to this comprehensive guide from IBM.
Strategies for Mitigating AI Bias
Several strategies can be employed to mitigate AI bias, ensuring fair and ethical AI systems. These strategies include:
- Inclusive and Diverse Training Data: Ensuring that training data represents a wide range of demographics can help in reducing bias. Companies like Microsoft are leading the way in promoting diverse datasets.
- Bias Detection and Mitigation Tools: Utilizing tools designed to detect and mitigate bias during the AI development process. Google’s Responsible AI Practices provide valuable resources for this purpose.
- Transparency and Explainability: Developing transparent AI models that can explain their decision-making process is critical. The World Economic Forum emphasizes the importance of explainable AI in ethical AI deployment.
Success Stories and Exemplary Cases
Numerous organizations have successfully implemented strategies to reduce AI bias, setting benchmarks for others to follow. Below are some exemplary cases:
- IBM: IBM has been at the forefront of addressing AI bias. Their Watson OpenScale platform is designed to detect and mitigate bias in AI models.
- Accenture: Accenture’s Responsible AI framework focuses on developing and deploying AI systems responsibly and ethically.
- Google: Google’s AI for Social Good initiative aims to leverage AI to address global challenges while ensuring fairness and reducing bias.
Practical Approaches to Reducing AI Bias
Implementing practical approaches is essential for reducing AI bias in real-world applications. Here are some effective methods:
Approach | Description | Example |
---|---|---|
Algorithmic Fairness | Developing algorithms that promote fairness by design. | Microsoft Fairlearn |
Bias Audits | Conducting regular audits of AI systems to identify and rectify biases. | O’Reilly’s Guide |
Human-in-the-Loop | Involving human oversight in AI decision-making processes to ensure fairness. | Accenture |
The Role of Policy and Regulation
Government policies and regulations play a critical role in mitigating AI bias. The European Union’s AI Regulation framework is a significant step towards ensuring ethical AI development and deployment. Similarly, the U.S. AI Bill of Rights outlines principles to protect individuals from AI-related harms.
Educational Initiatives and Awareness
Raising awareness and educating stakeholders about AI bias is vital. Initiatives such as Elements of AI offer free courses to the public, enhancing understanding of AI and its ethical implications.
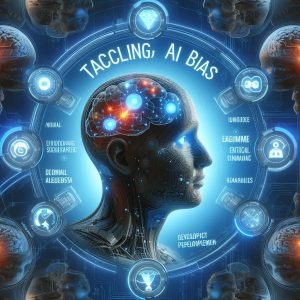
Conclusion
Tackling AI bias requires a multifaceted approach, involving inclusive training data, robust bias detection tools, transparent AI models, and comprehensive policies. By learning from success stories and implementing practical strategies, we can develop and deploy AI systems that are fair, ethical, and beneficial for all.
Pros and Cons of Tackling AI Bias
Addressing AI bias is a critical aspect of developing ethical AI systems. However, this process involves a range of advantages and challenges. This section outlines the key pros and cons associated with tackling AI bias.
Pros of Tackling AI Bias
- Improved Fairness and Equity: Addressing AI bias ensures that AI systems treat all users fairly, promoting equity across different demographics. This helps in building trust in AI technologies and ensures that they are used ethically. For example, fair AI algorithms can help reduce discriminatory practices in hiring, lending, and law enforcement.
- Enhanced Accuracy and Performance: Bias in AI systems can lead to inaccurate predictions and decisions. By mitigating bias, AI models can achieve higher accuracy and better performance, leading to more reliable outcomes. Companies like Microsoft have shown that reducing bias can significantly improve the performance of their AI systems.
- Compliance with Regulations: Governments and regulatory bodies are increasingly focusing on AI ethics. Tackling AI bias helps organizations comply with legal and regulatory requirements, avoiding potential fines and legal issues. The European Union’s AI Regulation framework, for example, mandates fair and transparent AI systems.
- Enhanced Reputation and Trust: Organizations that prioritize ethical AI practices, including addressing bias, can enhance their reputation and build trust with customers, stakeholders, and the public. Transparent and fair AI practices can lead to increased user satisfaction and loyalty.
- Fostering Innovation: Addressing AI bias can drive innovation by encouraging the development of new tools and methodologies for detecting and mitigating bias. This can lead to advancements in AI technology and its applications across various domains.
Cons of Tackling AI Bias
- Resource Intensive: Addressing AI bias requires significant resources, including time, money, and expertise. Organizations may need to invest in new technologies, hire specialists, and conduct extensive research and testing, which can be costly and time-consuming.
- Complexity and Technical Challenges: Mitigating AI bias is a complex task that involves understanding and addressing biases in data, algorithms, and deployment environments. It requires advanced technical skills and a deep understanding of both AI and the domain in which it is applied. This complexity can pose significant challenges for organizations.
- Unintended Consequences: Efforts to mitigate bias can sometimes lead to unintended consequences, such as overcompensation or new forms of bias. For example, in trying to balance representation, an AI system might inadvertently introduce bias against other groups.
- Impact on Performance: While addressing bias can improve fairness, it can also impact the performance of AI systems. In some cases, efforts to reduce bias might lead to trade-offs in accuracy or efficiency, especially if the bias mitigation techniques are not well-implemented.
- Resistance to Change: Organizations might face resistance from stakeholders who are accustomed to existing practices and systems. Implementing bias mitigation strategies may require cultural and organizational changes, which can be met with resistance and reluctance to adopt new approaches.
Balancing the Pros and Cons
While the challenges associated with tackling AI bias are significant, the benefits far outweigh the drawbacks. Organizations must weigh the pros and cons and develop comprehensive strategies to address AI bias effectively. Here are some balanced approaches:
- Invest in Education and Training: Educating AI developers, data scientists, and other stakeholders about AI bias and ethical AI practices is crucial. Training programs and workshops can help build the necessary skills and awareness to tackle bias effectively.
- Adopt a Multi-disciplinary Approach: Tackling AI bias requires collaboration between technologists, domain experts, ethicists, and policymakers. A multi-disciplinary approach can provide diverse perspectives and expertise, leading to more comprehensive and effective solutions.
- Utilize Bias Detection and Mitigation Tools: Leveraging advanced tools and technologies designed for bias detection and mitigation can streamline the process. Platforms like IBM Watson OpenScale and Google’s Responsible AI Practices offer valuable resources for addressing AI bias.
- Implement Continuous Monitoring and Auditing: Regular monitoring and auditing of AI systems are essential to identify and address biases that may emerge over time. Continuous evaluation ensures that AI systems remain fair and ethical throughout their lifecycle.
- Engage with the Community: Engaging with the broader community, including users, advocacy groups, and regulators, can provide valuable feedback and insights. Open dialogues and collaborations can help identify potential biases and develop more inclusive AI systems.
Conclusion
Tackling AI bias is a complex but necessary endeavor for developing ethical AI systems. While there are challenges and potential drawbacks, the benefits of improved fairness, accuracy, compliance, and reputation make it a worthwhile pursuit. By adopting balanced and strategic approaches, organizations can effectively mitigate AI bias and contribute to the development of ethical and trustworthy AI technologies.
Frequently Asked Questions (FAQs) on Tackling AI Bias
Addressing AI bias is a critical issue in the development and deployment of AI systems. Here are some frequently asked questions (FAQs) that provide insights and guidance on this topic.
What is AI bias?
AI bias refers to the systematic and unfair discrimination in the outcomes produced by AI systems. This bias can stem from biased training data, flawed algorithms, or the context in which the AI system is deployed. For more details, you can refer to this article by IBM.
Why is AI bias a problem?
AI bias can lead to unfair treatment of individuals or groups, perpetuating existing inequalities and creating new forms of discrimination. It can undermine trust in AI systems, lead to inaccurate and unreliable outcomes, and result in legal and ethical issues. For instance, biased AI in hiring processes can unfairly disadvantage certain demographic groups.
How does AI bias occur?
AI bias can occur at various stages, including data collection, algorithm design, and deployment. Bias in data arises when the training data is not representative of the real-world population or contains historical prejudices. Algorithmic bias occurs when the model’s design or assumptions introduce bias. Deployment bias happens when the AI system is applied in contexts that exacerbate existing biases. The Google AI Blog offers insights into these biases.
What are some strategies to mitigate AI bias?
Several strategies can help mitigate AI bias:
- Diverse and Inclusive Data: Ensure that training data is representative of diverse populations to reduce biases. This involves collecting data from various demographic groups and avoiding historical prejudices.
- Bias Detection Tools: Utilize tools and frameworks designed to detect and mitigate bias during the AI development process. Examples include Microsoft’s Fairlearn and Google’s AI Fairness 360.
- Transparency and Explainability: Develop AI models that are transparent and can explain their decision-making processes. This helps in identifying and addressing biases.
- Regular Audits: Conduct regular audits of AI systems to identify and rectify biases that may emerge over time. Continuous monitoring is essential to maintain fairness.
- Human Oversight: Incorporate human-in-the-loop systems where human judgment can intervene in AI decision-making processes to ensure fairness and accuracy.
Can AI systems be completely unbiased?
Achieving complete unbiasedness in AI systems is challenging due to the complexity of human societies and the inherent biases present in data and algorithms. However, it is possible to significantly reduce bias and its impact through diligent efforts in data collection, algorithm design, and continuous monitoring. Striving for minimal bias and transparency in AI systems is essential for ethical AI development.
How can organizations ensure their AI systems are fair?
Organizations can take several steps to ensure their AI systems are fair:
- Adopt Ethical AI Principles: Establish and adhere to ethical AI principles that prioritize fairness, transparency, and accountability. Organizations like Accenture have developed frameworks for responsible AI.
- Diverse Teams: Ensure that AI development teams are diverse and inclusive. Diverse teams can provide various perspectives and help identify potential biases early in the development process.
- Stakeholder Engagement: Engage with stakeholders, including users, advocacy groups, and regulators, to gather feedback and insights on AI system performance and fairness.
- Bias Mitigation Techniques: Implement bias mitigation techniques such as re-sampling, re-weighting, and algorithmic adjustments to reduce biases in AI models.
- Regular Training: Provide ongoing training and education for AI developers and data scientists on ethical AI practices and bias mitigation strategies.
What role do regulations play in addressing AI bias?
Regulations play a crucial role in addressing AI bias by setting standards and guidelines for fair and ethical AI development. Regulatory frameworks such as the European Union’s AI Regulation and the U.S. AI Bill of Rights outline principles and requirements for AI fairness, transparency, and accountability. These regulations help ensure that AI systems are developed and deployed responsibly, protecting individuals from AI-related harms.
What are some real-world examples of AI bias?
There have been several high-profile instances of AI bias in real-world applications:
- Facial Recognition: Facial recognition systems have been found to exhibit racial and gender biases, leading to higher error rates for certain demographic groups. For example, a study by the National Institute of Standards and Technology (NIST) highlighted significant disparities in facial recognition accuracy across different populations.
- Hiring Algorithms: Some hiring algorithms have been shown to favor certain candidates over others based on biased training data. For instance, Amazon discontinued an AI recruitment tool after discovering it was biased against female candidates.
- Loan Approval: AI systems used for loan approvals have faced scrutiny for discriminating against minority applicants. A study by the Brookings Institution discusses how algorithmic biases can lead to unfair lending practices.
How can bias be detected in AI systems?
Bias in AI systems can be detected using various techniques:
- Statistical Analysis: Analyzing the outcomes of AI models to identify disparities across different demographic groups can reveal biases. Metrics such as disparate impact and fairness indicators are useful for this purpose.
- Fairness Testing Tools: Utilizing specialized tools and frameworks designed for fairness testing, such as Microsoft Fairlearn and
Disclaimer and Caution
The content provided in this article on tackling AI bias is intended for informational purposes only. While we strive to provide accurate and up-to-date information, readers are cautioned to consider the following points when interpreting and applying the information discussed herein.
General Information
The information presented in this article is based on research and sources available at the time of writing. However, the field of artificial intelligence (AI) and bias mitigation is rapidly evolving, and new findings, tools, and methodologies may emerge that could impact the relevance and accuracy of the content. Readers are encouraged to consult multiple sources and stay informed about the latest developments in AI ethics and bias mitigation.
Not Professional Advice
This article does not constitute professional advice. The strategies, techniques, and tools discussed are general recommendations and may not be suitable for all contexts or applications. Organizations and individuals should seek professional guidance tailored to their specific needs and circumstances. Consulting with AI ethics experts, legal advisors, and other relevant professionals is recommended when developing and deploying AI systems.
Limitations and Assumptions
The discussion of AI bias mitigation strategies in this article is based on certain assumptions and limitations. The effectiveness of these strategies can vary depending on factors such as the quality and diversity of the data, the design and implementation of the algorithms, and the specific application context. Readers should critically assess these factors and consider the unique characteristics of their AI systems when applying the discussed strategies.
Unintended Consequences
Efforts to mitigate AI bias can sometimes lead to unintended consequences. For example, overcompensation in addressing one type of bias might introduce new biases or adversely affect other demographic groups. Additionally, bias mitigation techniques may impact the performance and accuracy of AI systems. It is important to continuously monitor and evaluate AI systems to identify and address any emerging issues.
Dynamic and Context-Specific Nature of AI Bias
AI bias is a dynamic and context-specific issue. Biases can manifest differently across various applications and user groups. What works to mitigate bias in one context may not be effective in another. Therefore, it is crucial to adopt a context-sensitive approach to bias mitigation, considering the specific needs, characteristics, and potential impacts on different stakeholders and applications.
Ethical and Legal Considerations
Addressing AI bias involves navigating complex ethical and legal considerations. Ethical principles such as fairness, transparency, and accountability should guide the development and deployment of AI systems. Additionally, organizations must comply with relevant laws and regulations governing AI ethics and bias mitigation. These regulations may vary across jurisdictions, and it is important to stay informed about and adhere to the applicable legal requirements.
Diverse Perspectives and Stakeholder Engagement
Effectively tackling AI bias requires diverse perspectives and stakeholder engagement. Collaboration between technologists, ethicists, domain experts, and affected communities is essential to identify and address biases comprehensively. Engaging with stakeholders, including users, advocacy groups, and regulators, can provide valuable insights and feedback, contributing to more inclusive and fair AI systems.
Continuous Improvement and Monitoring
Bias mitigation is not a one-time effort but an ongoing process. AI systems should be continuously monitored and audited to detect and address biases that may emerge over time. Regular evaluations, updates, and improvements are necessary to maintain the fairness and ethical integrity of AI systems. Organizations should establish robust mechanisms for ongoing monitoring, auditing, and stakeholder feedback.
Limitations of Bias Detection Tools
While various bias detection and mitigation tools are available, they have their limitations. These tools may not capture all forms of bias or may introduce new challenges. It is important to use these tools as part of a broader strategy that includes human judgment and oversight. Combining automated tools with human expertise can enhance the effectiveness of bias mitigation efforts.
Importance of Transparent Communication
Transparent communication about the presence and efforts to mitigate AI bias is crucial. Organizations should communicate openly with stakeholders about the limitations, challenges, and steps taken to address bias in their AI systems. Transparency builds trust and accountability, ensuring that users and other stakeholders are aware of the efforts to develop fair and ethical AI technologies.
Final Thoughts
The pursuit of fair and ethical AI is a complex but necessary endeavor. While there are challenges and uncertainties, the commitment to addressing AI bias can lead to more equitable and trustworthy AI systems. Readers are encouraged to stay informed, seek professional guidance, and actively participate in the ongoing efforts to develop and deploy ethical AI technologies.