From Pixels to Patterns: Understanding the Basics of Computer Vision
Computer vision, a subfield of artificial intelligence (AI), involves enabling computers to interpret and make decisions based on visual data. This technology has revolutionized numerous industries, from healthcare to automotive, by allowing machines to process and analyze images and videos at an unprecedented scale. In this article, we’ll delve into the basics of computer vision, exploring its key components, applications, and future trends.
Key Components of Computer Vision
At the core of computer vision are several fundamental processes that enable machines to understand visual data:
- Image Acquisition: Capturing visual data through cameras or sensors.
- Image Processing: Enhancing and manipulating images to highlight important features.
- Feature Extraction: Identifying and isolating significant parts of an image.
- Pattern Recognition: Recognizing patterns and objects within the image.
- Machine Learning: Using algorithms to learn from and make predictions based on visual data.
Applications of Computer Vision
The applications of computer vision are vast and varied, transforming multiple sectors:
Industry | Application | Example |
---|---|---|
Healthcare | Medical Imaging | GE Healthcare |
Automotive | Autonomous Vehicles | Tesla |
Retail | Inventory Management | Amazon Go |
Agriculture | Crop Monitoring | John Deere |
Security | Surveillance | Hikvision |
Success Stories and Exemplary Cases
Numerous companies have emerged as trailblazers in the field of computer vision, rewriting the norms of various industries and inspiring innovation. Here are some notable examples:
Waymo: A leader in autonomous driving technology, Waymo utilizes computer vision to navigate and understand the environment around its self-driving cars. Learn more about Waymo here.
DeepMind: Acquired by Google, DeepMind has made significant strides in healthcare using computer vision to analyze medical images and improve diagnostics. Discover more about DeepMind’s work here.
OpenAI: Known for its advancements in AI, OpenAI uses computer vision in various applications, including robotics and game playing. Explore OpenAI’s innovations here.
Challenges in Computer Vision
Despite its advancements, computer vision faces several challenges:
- Data Quality: Ensuring high-quality, annotated data for training models is crucial.
- Complexity: Dealing with complex, real-world environments requires sophisticated algorithms.
- Scalability: Scaling computer vision solutions to handle large datasets and diverse applications.
- Ethical Concerns: Addressing privacy issues and biases in visual data processing.
Future Trends in Computer Vision
The future of computer vision is promising, with several trends shaping its evolution:
- Integration with AI: Combining computer vision with other AI technologies to create more powerful solutions.
- Edge Computing: Processing visual data on devices closer to where it is collected, reducing latency.
- 3D Vision: Moving from 2D to 3D vision for more accurate object recognition and spatial understanding.
- Augmented Reality (AR): Enhancing real-world environments with computer-generated visual information.
- Sustainability: Developing energy-efficient computer vision systems to minimize environmental impact.
Conclusion
Computer vision is a transformative technology with the potential to revolutionize various industries. From enhancing healthcare diagnostics to enabling autonomous vehicles, its applications are vast and varied. While challenges remain, ongoing advancements in AI and machine learning promise to overcome these hurdles, paving the way for a future where machines can see and understand the world as humans do
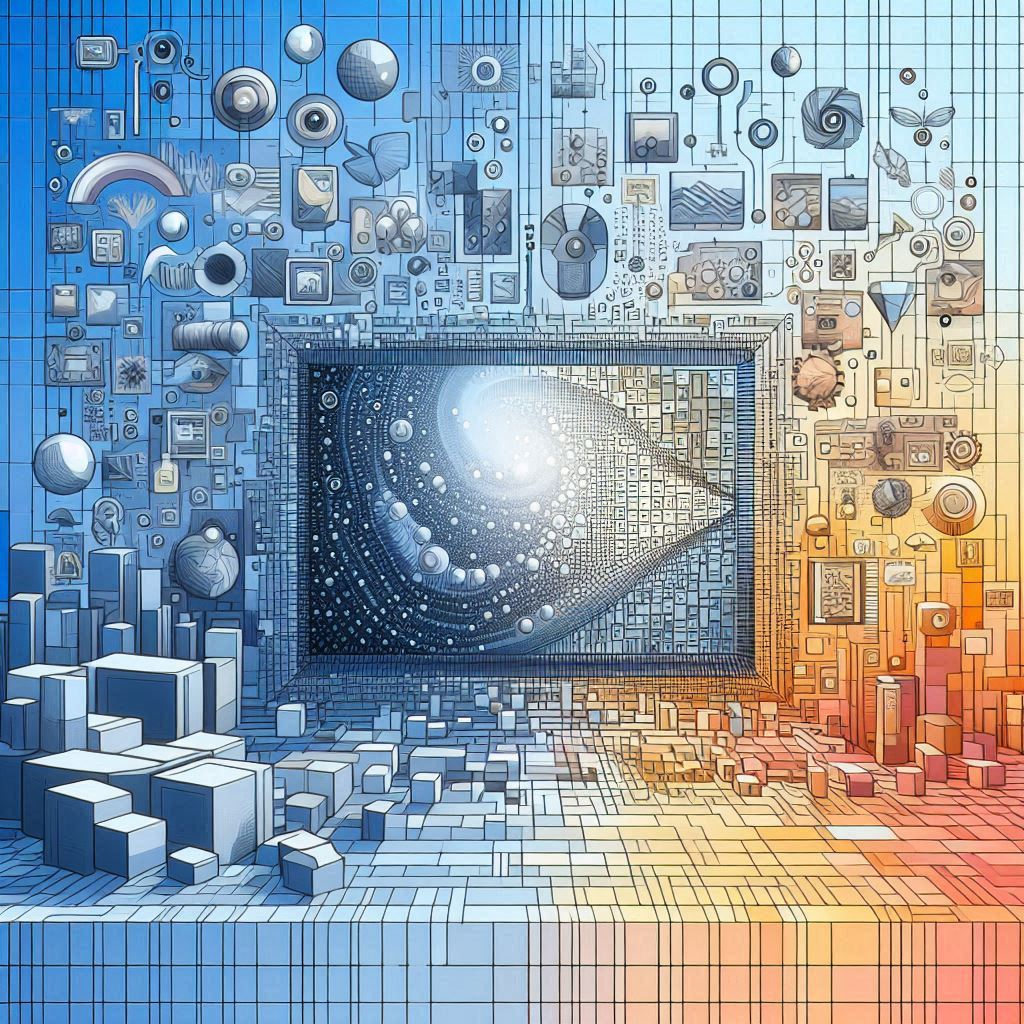