Building Trust in AI Ethics, Bias, and Responsible Innovation
Understanding AI’s Impact on Society
Artificial Intelligence (AI) has revolutionized industries, promising efficiency, innovation, and new possibilities across sectors ranging from healthcare to finance. However, as AI becomes more pervasive, concerns about ethics and bias have emerged as critical considerations in its development and deployment.
Ethics in AI involves ensuring that the technology respects fundamental human values and rights, operates transparently, and is accountable for its decisions and actions. Bias, on the other hand, refers to systematic errors or prejudices in AI systems that can result in unfair outcomes, perpetuate societal inequalities, or reinforce existing biases in data.
Ethical Frameworks and Guidelines
To address these challenges, various organizations and initiatives have developed ethical frameworks and guidelines for AI. The IEEE Global Initiative on Ethics of Autonomous and Intelligent Systems, for instance, outlines principles that emphasize human rights, transparency, and accountability in AI development and deployment. Similarly, the AI Ethics Guidelines Global Inventory provides a comprehensive database of AI ethics guidelines from around the world.
These frameworks aim to ensure that AI technologies are developed and used in ways that align with societal values and do not harm individuals or communities. They encourage developers and organizations to consider ethical implications throughout the AI lifecycle, from design and training to deployment and monitoring.
Bias Detection and Mitigation Strategies
Addressing bias in AI is crucial to building trust and ensuring fairness. Bias can manifest in various forms, including gender bias, racial bias, and socioeconomic bias, among others. Companies and researchers are actively developing tools and techniques to detect and mitigate bias in AI algorithms.
For example, IBM has developed the AI Fairness 360 toolkit, an open-source library that helps developers detect and mitigate bias in machine learning models. The toolkit includes algorithms and metrics for assessing fairness across different demographic groups and provides developers with tools to mitigate bias during the AI development process.
Similarly, Microsoft has integrated fairness considerations into its AI development practices through initiatives like Fairlearn. Fairlearn provides developers with tools and libraries to measure and mitigate unfairness in AI models, promoting more equitable outcomes in AI applications.
Success Stories and Exemplary Cases
Numerous organizations are leading by example in integrating ethical considerations into AI innovation. Companies like IBM and Microsoft are at the forefront of promoting responsible AI practices through their initiatives and technologies.
Company | Initiative | Impact |
---|---|---|
IBM | AI Fairness 360 Toolkit | Helps developers detect and mitigate bias in AI models, promoting fairness and transparency. |
Microsoft | Fairlearn | Provides tools and libraries to measure and mitigate unfairness in AI models, ensuring more equitable outcomes. |
These initiatives demonstrate a commitment to building trustworthy AI systems that benefit society while minimizing harm and ensuring fairness for all users.
Challenges and Future Directions
Despite significant progress, several challenges remain in the quest to build trust in AI. Regulatory frameworks often lag behind technological advancements, making it difficult to enforce ethical standards uniformly across jurisdictions. Additionally, the complexity of AI systems and the potential for unintended consequences pose ongoing challenges for developers and policymakers alike.
Looking ahead, there is a need for continued research and innovation in AI ethics, bias detection, and mitigation strategies. Collaboration among stakeholders—including governments, industry leaders, researchers, and civil society organizations—is essential to address these complex issues effectively and ensure that AI technologies are developed and deployed responsibly.
Conclusion
Building trust in AI requires a concerted effort to integrate ethical considerations, detect and mitigate bias, and foster transparency and accountability throughout the AI lifecycle. By doing so, we can harness the transformative potential of AI while safeguarding fundamental rights and values.
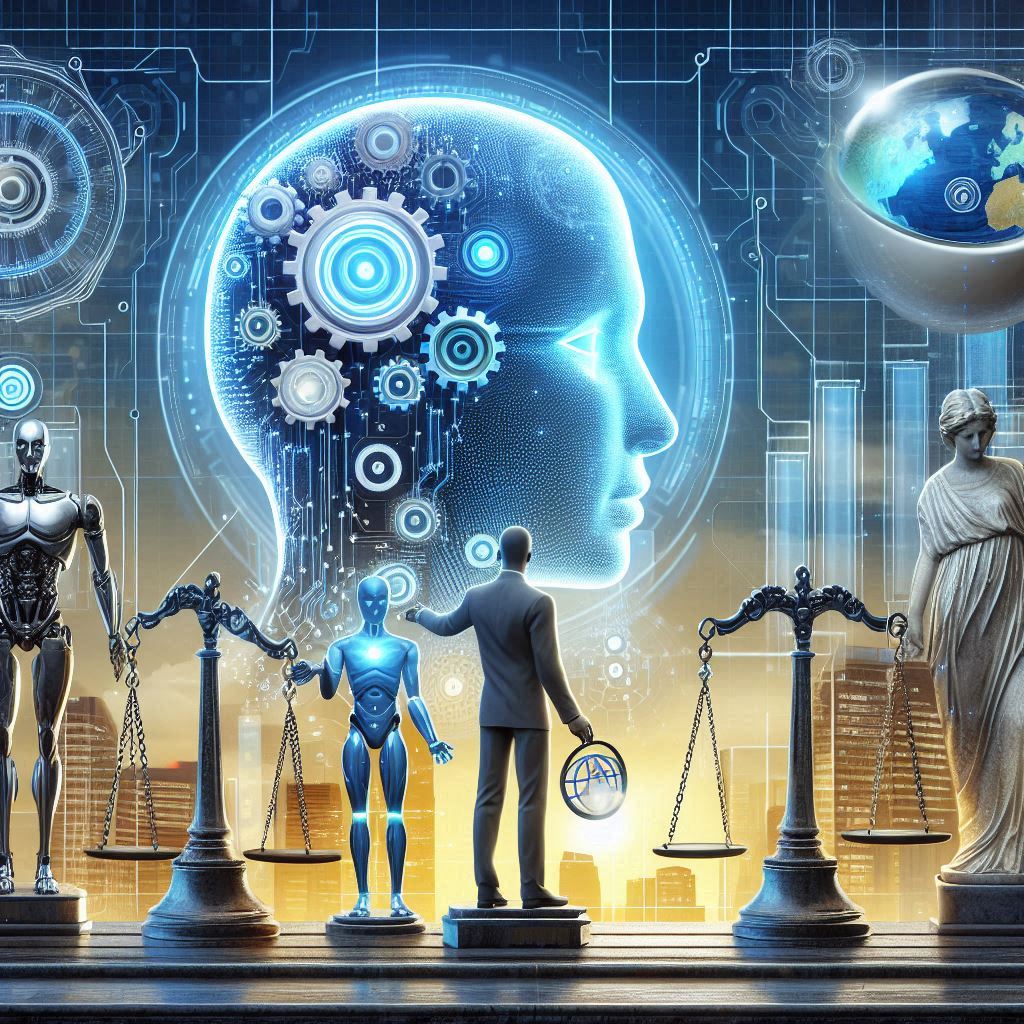
Additional Resources
For further reading on AI ethics and responsible innovation, explore the resources provided by the IEEE Global Initiative on Ethics of Autonomous and Intelligent Systems and the AI Ethics Guidelines Global Inventory.
This expanded version includes more detailed sections, a table highlighting success stories, and a comprehensive exploration of the ethical, bias-related, and innovative aspects of AI trust-building. Adjustments can be made based on specific requirements or additional information you’d like to include.
Building Trust in AI: Pros and Cons
Artificial Intelligence (AI) is rapidly transforming industries and everyday life, promising efficiency, innovation, and new opportunities. However, the widespread adoption of AI comes with significant challenges, particularly concerning ethics, bias, and responsible innovation. Building trust in AI systems is crucial to ensuring their acceptance and beneficial impact on society. This article explores the pros and cons of efforts to build trust in AI through ethical frameworks, bias detection strategies, and responsible innovation.
I. Pros of Building Trust in AI
A. Ethical Frameworks
Ethical frameworks play a crucial role in guiding the development and deployment of AI technologies. These frameworks are designed to ensure that AI systems operate transparently, respect fundamental human values and rights, and are accountable for their decisions and actions. By adhering to ethical guidelines, organizations can mitigate risks associated with AI deployment and build trust among users and stakeholders.
Definition and Purpose: Ethical frameworks provide a structured approach to addressing ethical challenges in AI, such as fairness, transparency, accountability, and privacy. They set standards for developers and organizations to follow throughout the AI lifecycle—from data collection and model training to deployment and monitoring.
Ensuring Transparency and Accountability: Ethical frameworks promote transparency by requiring organizations to disclose how AI systems operate, including their data sources, algorithms, and decision-making processes. This transparency fosters trust among users by enabling them to understand and verify AI systems’ behavior.
Upholding Societal Values and Rights: AI ethical guidelines emphasize the importance of respecting human rights and societal values. By prioritizing ethical considerations, organizations can develop AI applications that benefit individuals and communities while minimizing harm and potential negative impacts.
Examples of Successful Implementation: Initiatives such as the IEEE Global Initiative on Ethics of Autonomous and Intelligent Systems and the AI Ethics Guidelines Global Inventory provide comprehensive frameworks and guidelines for ethical AI development. These initiatives showcase successful implementation of ethical principles across diverse sectors and regions, demonstrating their practical relevance and impact.
B. Bias Detection Strategies
Addressing bias in AI algorithms is critical to ensuring fairness and mitigating potential harm to individuals and communities. Bias can arise from various sources, including data collection practices, algorithm design choices, and societal biases embedded in training datasets. Effective bias detection strategies help identify and mitigate biases, promoting equitable outcomes and enhancing trust in AI systems.
Importance of Addressing Bias: Bias in AI can perpetuate existing inequalities and lead to unfair treatment across demographic groups. Detecting and mitigating bias is essential to building inclusive AI systems that deliver equitable outcomes for all users.
Techniques and Tools for Bias Detection: Companies and researchers have developed various techniques and tools to detect and mitigate bias in AI models. For example, the AI Fairness 360 toolkit developed by IBM provides developers with algorithms and metrics to assess and mitigate bias across different demographic groups.
Mitigating Unfair Outcomes: Bias detection strategies help identify instances where AI systems produce unfair outcomes and enable developers to adjust algorithms or data inputs to minimize bias. By promoting fairness and equity, these strategies enhance trust in AI applications and foster greater acceptance among users.
Case Studies of Effective Bias Detection and Mitigation: Organizations like IBM and Microsoft have implemented successful bias detection and mitigation strategies in their AI development practices. These initiatives demonstrate how proactive measures can address bias and contribute to building trust in AI technologies.
C. Responsible Innovation
Responsible innovation involves integrating ethical considerations into the design, development, and deployment of AI technologies. It aims to minimize risks and maximize benefits associated with AI adoption, ensuring that technological advancements contribute positively to societal well-being and economic development.
Integrating Ethics from Design to Deployment: Responsible innovation emphasizes the importance of considering ethical implications throughout the AI lifecycle. By integrating ethics into the design phase, developers can anticipate potential ethical challenges and proactively address them during development and deployment.
Minimizing Unintended Consequences: AI systems can have unintended consequences, such as privacy violations, job displacement, or reinforcement of societal biases. Responsible innovation seeks to mitigate these risks through careful planning, stakeholder engagement, and ongoing evaluation of AI systems’ impacts.
Promoting Innovation for Social Good: Responsible innovation encourages the development of AI technologies that benefit society and contribute to sustainable development goals. By focusing on social impact and ethical integrity, organizations can enhance public trust and confidence in AI applications.
Success Stories in Responsible AI Innovation: Industries such as healthcare, finance, and transportation have witnessed transformative advancements through responsible AI innovation. For example, AI-powered medical diagnostics improve patient outcomes, while ethical AI in finance promotes fairness and transparency in decision-making processes.
II. Cons of Building Trust in AI
While efforts to build trust in AI through ethical frameworks, bias detection strategies, and responsible innovation offer significant benefits, several challenges and limitations must be considered.
A. Challenges in Ethical Frameworks
Ethical frameworks face several challenges that can hinder their effectiveness and adoption across industries and regions.
Lack of Universal Standards: The absence of universal ethical standards for AI development and deployment complicates efforts to ensure consistent ethical practices globally. Varying regulatory frameworks and cultural norms further contribute to uncertainty and ambiguity in ethical guidelines.
Balancing Innovation with Regulatory Compliance: Ethical guidelines must strike a balance between fostering innovation and ensuring regulatory compliance. Strict regulatory requirements may stifle technological advancements, while lenient regulations could lead to ethical lapses and public mistrust.
Ethical Dilemmas in AI Decision-Making: AI systems often face ethical dilemmas, such as prioritizing competing values or making decisions with uncertain consequences. Resolving these dilemmas requires nuanced ethical reasoning and may not always result in universally acceptable outcomes.
Public Skepticism and Trust Deficits: Despite efforts to promote ethical AI practices, public skepticism and distrust in AI technologies persist. High-profile incidents of AI misuse or ethical breaches contribute to public apprehension and reluctance to embrace AI-driven solutions.
B. Limitations of Bias Detection Strategies
While bias detection strategies are essential for promoting fairness in AI systems, they are not without limitations and challenges.
Complexity in Detecting Subtle Biases: Identifying subtle forms of bias, such as implicit biases embedded in data or algorithmic decisions, poses significant challenges for developers and researchers. Current bias detection techniques may overlook nuanced biases that impact AI performance.
Bias in Data Collection and Preprocessing: Biases can originate from biased data sources or inadequate preprocessing techniques. Ensuring data quality and diversity is essential for reducing biases in AI models but requires comprehensive data governance and management practices.
Algorithmic Limitations: AI algorithms may exhibit limitations in detecting and mitigating bias effectively. Issues such as algorithmic bias, where algorithms systematically favor certain groups over others, necessitate continuous refinement and validation of bias detection methods.
Effectiveness Across Diverse Demographic Groups: Bias detection strategies must account for diversity within user populations and ensure equitable outcomes across demographic groups. Addressing intersectional biases—biases that affect individuals based on multiple characteristics—is particularly challenging but essential for promoting inclusivity in AI applications.
C. Issues with Responsible Innovation
Responsible innovation in AI faces several barriers and challenges that impact its implementation and scalability.
Pace of Technological Advancements vs. Regulatory Adaptation: Rapid advancements in AI technology outpace regulatory frameworks’ ability to adapt and respond effectively. This discrepancy creates uncertainty and regulatory lag, hindering responsible innovation initiatives.
Resistance to Change in Established Industries: Industries resistant to change may prioritize profit motives over ethical considerations, leading to ethical dilemmas and public backlash. Overcoming institutional inertia requires leadership commitment and industry-wide collaboration.
Cost and Resource Implications for SMEs: Small and medium enterprises (SMEs) may lack the resources and expertise needed to implement responsible AI practices effectively. Cost considerations, including investment in AI ethics training and infrastructure, pose barriers to SME participation in responsible innovation.
Ethical Challenges in Emerging AI Applications: Emerging AI applications, such as autonomous vehicles, predictive policing, and facial recognition technologies, present unique ethical challenges. These applications raise concerns about privacy, surveillance, and societal impact, necessitating careful consideration of ethical implications and stakeholder perspectives.
Frequently Asked Questions (FAQs) on Building Trust in AI
1. What are ethical frameworks in AI, and why are they important?
Ethical frameworks in AI refer to guidelines and principles that govern the development and deployment of AI technologies. They are essential for ensuring that AI systems operate ethically, transparently, and accountably. Ethical frameworks help address concerns such as fairness, privacy, accountability, and transparency, promoting trust among users and stakeholders. By adhering to ethical guidelines, organizations can mitigate risks associated with AI deployment and foster responsible innovation that aligns with societal values and expectations.
2. How do ethical frameworks contribute to building trust in AI?
Ethical frameworks contribute to building trust in AI by providing clear standards and principles for developers and organizations to follow. They promote transparency by requiring AI systems to disclose how they operate, including their data sources, algorithms, and decision-making processes. This transparency enables users to understand and verify AI systems’ behavior, thereby increasing trust and confidence in their reliability and fairness. Ethical frameworks also emphasize accountability, ensuring that organizations are held responsible for the ethical implications of their AI technologies.
3. What are some examples of successful implementation of ethical frameworks in AI?
Several organizations and initiatives have successfully implemented ethical frameworks in AI development and deployment. For example, the IEEE Global Initiative on Ethics of Autonomous and Intelligent Systems provides guidelines that emphasize human rights, transparency, and accountability in AI technologies. Similarly, the AI Ethics Guidelines Global Inventory offers a comprehensive database of AI ethics guidelines from around the world, showcasing diverse approaches to ethical AI development. Companies like IBM and Microsoft integrate ethical considerations into their AI practices through initiatives such as the AI Fairness 360 toolkit and Fairlearn, promoting fairness and transparency in AI applications.
4. Why is bias detection important in AI, and how does it enhance trust?
Bias detection in AI is crucial for identifying and mitigating biases that can lead to unfair or discriminatory outcomes. Bias can arise from various sources, including biased data collection practices, algorithmic design choices, and societal prejudices embedded in training datasets. Detecting and mitigating bias helps ensure that AI systems produce equitable outcomes for all users, regardless of demographic characteristics. By addressing bias, organizations can enhance trust in AI systems by demonstrating their commitment to fairness, inclusivity, and ethical integrity.
5. What techniques and tools are used for bias detection in AI?
Various techniques and tools are employed for bias detection in AI, ranging from statistical analysis to machine learning algorithms. Common approaches include:
Statistical Analysis: Analyzing dataset distributions to identify disparities across demographic groups.
Algorithmic Audits: Evaluating AI models to detect patterns of bias in decision-making processes.
Fairness Metrics: Developing metrics to assess fairness in AI outputs, such as disparate impact analysis and fairness-aware machine learning.
Bias Mitigation Strategies: Implementing techniques to adjust algorithms or data inputs to minimize bias and promote equitable outcomes.
Companies like IBM’s AI Fairness 360 toolkit and Microsoft’s Fairlearn provide developers with tools and libraries to detect and mitigate bias in AI models, enhancing trust and fairness in AI applications.
6. What are the limitations of bias detection strategies in AI?
While bias detection strategies are essential for promoting fairness in AI systems, they are not without limitations. Some challenges include:
Complexity in Detecting Subtle Biases: Identifying subtle forms of bias, such as implicit biases embedded in data or algorithmic decisions, can be challenging.
Bias in Data Collection and Preprocessing: Biases may originate from biased data sources or inadequate preprocessing techniques, affecting AI model performance.
Algorithmic Limitations: AI algorithms may exhibit biases or limitations in detecting and mitigating bias effectively, requiring continuous refinement and validation.
Effectiveness Across Diverse Demographic Groups: Bias detection strategies must account for diversity within user populations and ensure equitable outcomes across different demographic groups.
Addressing these limitations requires ongoing research, development, and collaboration among stakeholders to enhance the effectiveness and reliability of bias detection techniques in AI.
7. What is responsible innovation in AI, and why is it important?
Responsible innovation in AI refers to the integration of ethical considerations into the design, development, and deployment of AI technologies. It aims to minimize risks and maximize benefits associated with AI adoption, ensuring that technological advancements contribute positively to societal well-being and economic development. Responsible innovation promotes transparency, accountability, and sustainability in AI practices, fostering trust and confidence among users, policymakers, and the public.
8. How does responsible innovation contribute to building trust in AI?
Responsible innovation contributes to building trust in AI by addressing ethical concerns and minimizing potential harms associated with AI technologies. By integrating ethics from the outset, developers can anticipate and mitigate ethical risks throughout the AI lifecycle. Responsible innovation emphasizes stakeholder engagement, transparency, and accountability, ensuring that AI systems are developed and deployed in ways that align with societal values and expectations. By promoting ethical integrity and social responsibility, responsible innovation enhances trust in AI applications and facilitates their acceptance and adoption across diverse sectors and communities.
Disclaimer and Caution: Ethical Considerations, Bias, and Responsible Innovation in AI
Artificial Intelligence (AI) technologies are transforming industries and societies, offering new opportunities for innovation, efficiency, and societal advancement. However, the deployment of AI systems raises ethical considerations, potential biases, and challenges related to responsible innovation. This disclaimer and cautionary section aims to highlight key issues, challenges, and considerations associated with the use of AI, emphasizing the importance of ethical frameworks, bias detection strategies, and responsible innovation.
1. Ethical Considerations in AI
AI technologies have the potential to impact individuals, communities, and societies in profound ways. Ethical considerations in AI are critical for ensuring that these technologies are developed and deployed in a manner that respects human values, rights, and societal norms. Ethical frameworks provide guidelines and principles to guide developers, organizations, and policymakers in navigating ethical dilemmas and promoting responsible AI practices.
Transparency and Accountability: AI systems should operate transparently, with clear documentation of data sources, algorithms, and decision-making processes. Organizations must be accountable for the ethical implications of their AI technologies, ensuring that they align with societal values and expectations.
Fairness and Equity: AI systems should be designed to minimize biases and ensure fairness in decision-making processes. Bias detection strategies and fairness metrics play a crucial role in identifying and mitigating biases that may lead to unfair outcomes for individuals or groups.
Privacy and Data Protection: Protecting user privacy and data rights is essential in AI development and deployment. Organizations must adhere to data protection regulations and implement measures to safeguard sensitive information from unauthorized access or misuse.
Human-Centered Design: AI technologies should prioritize human well-being and autonomy. Human-centered design principles promote user-centric approaches that empower individuals and enhance their experiences with AI systems.
2. Challenges and Risks
Despite the potential benefits of AI technologies, their deployment presents various challenges and risks that require careful consideration and management:
Bias and Fairness: Bias can inadvertently be introduced into AI systems through biased data sources, algorithmic design choices, or unintended consequences. Detecting and mitigating bias requires robust techniques and ongoing monitoring to ensure equitable outcomes.
Regulatory and Legal Compliance: Adhering to regulatory requirements and legal frameworks is essential for mitigating risks associated with AI deployment. Organizations must navigate complex regulatory landscapes and ensure compliance with data protection, privacy, and anti-discrimination laws.
Ethical Dilemmas: AI technologies may present ethical dilemmas, such as prioritizing competing values or making decisions with uncertain consequences. Addressing these dilemmas requires ethical reasoning, stakeholder engagement, and transparent decision-making processes.
Public Trust and Perception: Maintaining public trust and confidence in AI technologies is crucial for their acceptance and adoption. High-profile incidents of AI misuse, ethical breaches, or unintended consequences can erode trust and contribute to skepticism about AI’s benefits and risks.
Societal Impact: AI technologies can have broad societal impacts, including job displacement, changes in socioeconomic dynamics, and implications for equity and social justice. Responsible innovation seeks to mitigate these impacts and maximize positive outcomes for society as a whole.
3. Responsible Innovation in AI
Responsible innovation in AI emphasizes ethical integrity, transparency, and accountability throughout the AI lifecycle—from design and development to deployment and evaluation. It aims to minimize risks and maximize benefits associated with AI adoption, ensuring that technological advancements contribute positively to societal well-being and economic development.
Integration of Ethical Considerations: Responsible innovation requires integrating ethical considerations into AI design and decision-making processes. Organizations should anticipate potential ethical challenges, engage stakeholders, and implement measures to promote ethical behavior and decision-making.
Stakeholder Engagement: Engaging diverse stakeholders, including users, communities, and policymakers, is essential for understanding and addressing societal concerns about AI technologies. Stakeholder input informs ethical guidelines, regulatory frameworks, and responsible AI practices.
Continuous Evaluation and Improvement: Responsible innovation involves ongoing evaluation and improvement of AI systems’ performance, impact, and adherence to ethical standards. Organizations should adopt a culture of continuous learning and adaptation to address emerging ethical issues and technological developments.
Sustainability and Social Responsibility: AI technologies should contribute positively to sustainable development goals and social responsibility. Responsible innovation promotes environmentally sustainable practices, equitable access to AI benefits, and inclusive economic growth.
Risk Management and Mitigation: Proactively identifying and managing risks associated with AI deployment is crucial for responsible innovation. Organizations should develop risk management strategies, contingency plans, and crisis management protocols to address potential ethical lapses or adverse impacts.
4. Cautionary Notes
While AI technologies offer significant potential benefits, users, developers, and organizations should approach their development and deployment with caution and mindfulness of ethical considerations, biases, and responsible innovation principles:
Ethical Diligence: Prioritize ethical considerations in AI development, deployment, and use to ensure alignment with societal values and ethical standards.
Bias Awareness: Be vigilant in detecting and mitigating biases that may affect AI systems’ fairness and accuracy in decision-making.
Regulatory Compliance: Adhere to applicable laws, regulations, and standards governing AI development, data protection, privacy, and non-discrimination.
Transparency and Accountability: Foster transparency by disclosing how AI systems operate, including their data inputs, algorithms, and decision outcomes. Hold organizations accountable for the ethical implications of their AI technologies.
Public Engagement: Engage with stakeholders, including users, communities, and policymakers, to understand and address concerns about AI technologies’ impact on society.
Continuous Improvement: Commit to continuous evaluation, learning, and improvement of AI systems’ performance, impact, and ethical integrity.
5. Conclusion
In conclusion, the development and deployment of AI technologies require careful consideration of ethical guidelines, bias detection strategies, and responsible innovation practices. By prioritizing ethical integrity, transparency, and accountability, organizations can build trust and confidence in AI systems while mitigating risks and maximizing benefits for individuals, communities, and society as a whole. It is essential for stakeholders to collaborate, innovate responsibly, and navigate ethical complexities to ensure that AI technologies contribute positively to global progress and human well-being.
This disclaimer and cautionary section provides a comprehensive overview of ethical considerations, challenges, and responsible innovation practices in AI. It aims to inform stakeholders about the importance of ethical integrity, bias detection, and responsible deployment of AI technologies while encouraging mindful and responsible innovation practices.