AI Ethics and Bias: Navigating the Challenges of Fairness and Accountability
Introduction
Artificial Intelligence (AI) is revolutionizing industries, driving innovation, and enhancing efficiency across various sectors. However, the increasing reliance on AI brings critical ethical challenges, particularly regarding fairness and accountability. AI systems can unintentionally perpetuate and amplify biases present in training data, leading to unfair and discriminatory outcomes. Addressing these issues is crucial for ensuring that AI technology benefits all members of society equitably.
Understanding AI Bias
AI bias occurs when an AI system produces results that are systematically prejudiced due to erroneous assumptions in the machine learning process. These biases can arise from various sources, including historical data, algorithm design, and human intervention. For instance, if an AI system is trained on data that reflects societal inequalities, it is likely to reproduce those biases in its predictions and decisions.
Types of Bias in AI
Type of Bias | Description | Example |
---|---|---|
Historical Bias | Arises from data that reflects past prejudices and inequalities. | Racial bias in criminal justice AI systems. |
Representation Bias | Occurs when certain groups are underrepresented in the training data. | Gender bias in facial recognition systems. |
Measurement Bias | Stems from inaccuracies in data collection or labeling. | Incorrect tagging of images in datasets. |
Challenges of Fairness in AI
Ensuring fairness in AI involves addressing complex and multifaceted challenges. One major issue is the lack of a universal definition of fairness, which can vary depending on cultural, legal, and social contexts. Additionally, achieving fairness often requires balancing competing values, such as accuracy versus equity. Furthermore, the dynamic nature of AI systems, which continuously learn and evolve, makes it difficult to maintain fairness over time.
Approaches to Mitigate AI Bias
Several strategies can help mitigate bias in AI systems:
- Data Auditing: Regularly examining and updating training data to ensure it is representative and free from bias.
- Algorithmic Fairness: Developing algorithms designed to identify and correct bias in predictions.
- Transparency: Implementing transparent AI models that allow for scrutiny and understanding of decision-making processes.
- Inclusive Design: Involving diverse teams in AI development to bring different perspectives and reduce bias.
Success Stories and Exemplary Cases
Numerous companies and organizations are making strides in addressing AI bias and promoting fairness:
- IBM: IBM’s AI Fairness 360 toolkit provides open-source resources to help developers detect and mitigate bias in AI models.
- Microsoft: Microsoft’s Fairness, Accountability, Transparency, and Ethics (FATE) group focuses on researching and developing tools to ensure ethical AI practices.
- Google: Google has implemented fairness measures in its AI systems, such as the What-If Tool, to analyze how models treat different demographic groups.
Accountability in AI
Ensuring accountability in AI systems involves establishing clear responsibility for the outcomes produced by these technologies. This requires transparency in AI development and deployment processes, as well as robust mechanisms for auditing and oversight. Accountability also involves the ability to explain AI decisions, allowing stakeholders to understand how outcomes are reached and to challenge unfair or harmful results.
Regulatory and Ethical Frameworks
Various regulatory and ethical frameworks aim to guide the responsible use of AI:
- EU AI Act: The European Union’s proposed AI regulation aims to ensure AI systems are safe and respect fundamental rights and values.
- OECD AI Principles: The Organisation for Economic Co-operation and Development has established principles to promote AI that is innovative, trustworthy, and respects human rights.
- IEEE Global Initiative: The Institute of Electrical and Electronics Engineers provides ethical guidelines for autonomous and intelligent systems to prioritize human well-being.
The Role of Stakeholders in Ensuring Ethical AI
Multiple stakeholders play a crucial role in promoting ethical AI:
- Developers: Responsible for designing and building AI systems with fairness and accountability in mind.
- Policymakers: Tasked with creating and enforcing regulations that ensure the ethical use of AI.
- Users: Need to be informed about the capabilities and limitations of AI systems to use them responsibly.
- Academics: Conduct research to identify and address ethical challenges in AI.
Case Studies in Ethical AI Implementation
Several case studies illustrate the successful implementation of ethical AI practices:
- Accenture: Accenture has developed a fairness tool to evaluate AI models for potential biases, ensuring fair outcomes in hiring and other processes.
- Better.com:
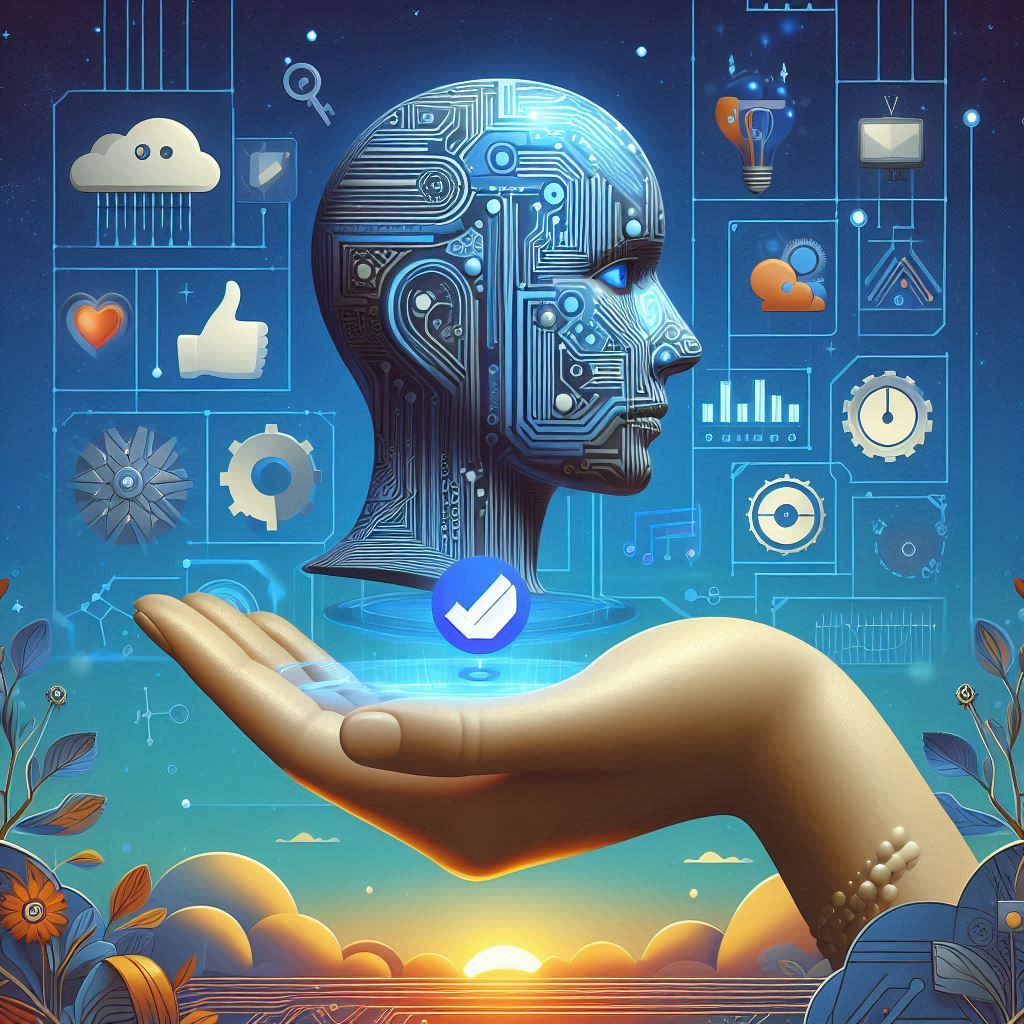