Advancements in Object Detection: Pushing the Boundaries of Computer Vision
In recent years, object detection has made significant strides, revolutionizing industries ranging from autonomous vehicles to healthcare. This article explores the cutting-edge technologies and success stories that are shaping the future of computer vision.
In recent years, object detection technology has emerged as a transformative force, reshaping industries and pushing the boundaries of computer vision capabilities. From autonomous vehicles navigating city streets to healthcare systems diagnosing diseases, the applications of object detection are diverse and impactful.
Advancements in Object Detection Technology
Advancements in Object Detection Technology
Object detection technology has evolved significantly, driven by advancements in deep learning algorithms, computational power, and data availability. Traditional approaches like sliding window and handcrafted feature extraction have given way to more sophisticated methods such as convolutional neural networks (CNNs) and region-based detectors like Faster R-CNN and Mask R-CNN. These advancements have enabled faster processing speeds and higher detection accuracy, making real-time applications feasible in dynamic environments.
Applications Across Industries
Autonomous Vehicles:
One of the most prominent applications of object detection is in autonomous vehicles. These vehicles rely on sensors and cameras equipped with object detection algorithms to detect and classify objects such as pedestrians, cyclists, and other vehicles in their vicinity. This capability is crucial for making real-time decisions to ensure passenger safety and efficient navigation.
Healthcare:
In healthcare, object detection plays a critical role in medical imaging analysis. Radiologists use object detection algorithms to identify and localize anomalies in X-rays, MRIs, and CT scans. This technology enhances diagnostic accuracy, speeds up treatment planning, and improves patient outcomes by enabling early detection of diseases.
Security and Surveillance:
Security systems leverage object detection to monitor public spaces, identify suspicious activities, and enhance situational awareness. Video surveillance cameras equipped with object detection algorithms can automatically detect intrusions, track individuals of interest, and alert security personnel in real-time, thereby improving overall security measures.
Retail and Customer Analytics:
In retail, object detection technology facilitates inventory management, customer behavior analysis, and personalized shopping experiences.e Retailers us CCTV cameras and smart sensors to track product movements, monitor store traffic patterns, and analyze customer demographics. This data-driven approach helps optimize inventory levels, enhance marketing strategies, and improve customer satisfaction.
Success Stories and Future Outlook
The success stories of object detection technology are manifold. Companies like Tesla have integrated advanced object detection systems into their autonomous driving platforms, setting new benchmarks for safety and efficiency in the automotive industry. Similarly, healthcare providers are adopting AI-driven medical imaging solutions to achieve faster diagnoses and more personalized treatment plans.
Looking ahead, the future of object detection technology holds promise for even greater advancements. Researchers are exploring new techniques such as 3D object detection, multi-modal sensor fusion, and improved model interpretability. These innovations aim to overcome current limitations and expand the scope of applications across diverse fields.
In conclusion, object detection technology represents a paradigm shift in computer vision, driving innovation and revolutionizing industries from automotive to healthcare. With continuous research and technological advancements, the potential for object detection to further enhance safety, efficiency, and decision-making capabilities is limitless, paving the way for a future where intelligent machines seamlessly interact with and interpret their surroundings.
Success Stories and Exemplary Cases
Object detection technologies have enabled remarkable achievements in various fields:
Company | Industry | Technology |
---|---|---|
Tesla | Automotive | Autonomous driving systems |
Microsoft | Technology | Edge AI applications |
IBM | Healthcare | Medical imaging analysis |
Technological Innovations and Breakthroughs
Recent advancements in object detection have been propelled by:
- NVIDIA‘s CUDA platform for accelerated deep learning
- Google’s TensorFlow framework for scalable model training
- PyTorch‘s dynamic computation graph for flexible neural network architectures
Challenges and Future Directions
Despite its successes, object detection faces challenges such as:
- Ensuring robustness against occlusions and varying lighting conditions
- Addressing ethical concerns related to privacy and data security
Conclusion
Object detection continues to redefine possibilities in computer vision, driving innovations across industries. With ongoing research and technological advancements, the future promises even more transformative applications.
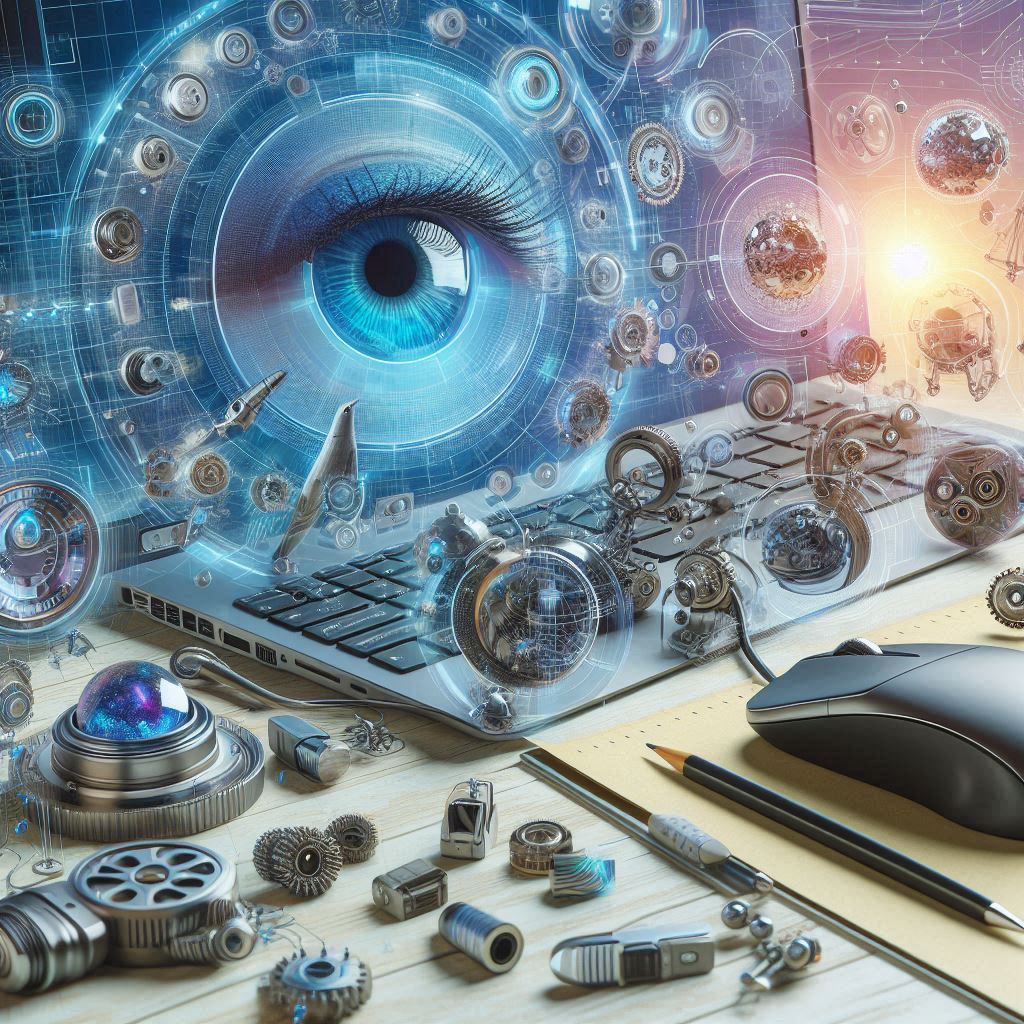
Outline: Pros and Cons of Object Detection Technology
Introduction
Brief overview of object detection technology
Importance in various industries like autonomous driving, healthcare, security, etc.
Pros of Object Detection Technology
Enhanced Safety and Security
Applications in surveillance systems, threat detection, and crime prevention.
Examples: Use in airports, public spaces for real-time monitoring.
Automation and Efficiency
Streamlining processes in industries like manufacturing and logistics.
Case studies: Robotics in assembly lines, warehouse management.
Advancements in Healthcare
Medical imaging analysis for diagnostics and treatment planning.
Success stories: Radiology and pathology applications.
Improved User Experience
Personalized services in retail and entertainment sectors.
Examples: Recommendation systems based on object detection data.
Support for Accessibility
Assistive technologies for visually impaired individuals.
Tools: Object recognition apps and devices.
Cons of Object Detection Technology
Privacy Concerns
Issues related to surveillance and data collection.
Case studies: Public debate over facial recognition technology.
Bias and Ethical Challenges
Algorithmic biases impacting decision-making processes.
Examples: Controversies in law enforcement and hiring practices.
Complexity and Cost
High implementation costs and technological dependencies.
Challenges: Maintenance and scalability issues in large-scale deployments.
Technical Limitations
Challenges in detecting occluded or overlapping objects.
Case studies: Limitations in adverse weather conditions.
Legal and Regulatory Issues
Compliance with data protection laws and ethical guidelines.
Examples: GDPR implications and regulatory frameworks.
Conclusion
Recap of the benefits and drawbacks of object detection technology.
Future outlook: Innovations and solutions to address current challenges.
This outline provides a structured approach to discuss the positive impacts and challenges associated with object detection technology, allowing for a comprehensive exploration in the full article.
FAQs: Object Detection Technology Explained
Introduction
Object detection technology has revolutionized numerous industries by enabling machines to perceive and understand their environments through visual data. This section addresses common questions to provide a deeper understanding of how object detection works, its applications, benefits, limitations, ethical considerations, and future advancements.
Frequently Asked Questions
What is object detection technology?
Object detection technology is a subset of computer vision that focuses on identifying and locating objects within digital images or videos. It involves techniques like image preprocessing, feature extraction, classification, and localization. Unlike image classification that identifies the entire image content, object detection pinpoints the presence and position of specific objects within an image, making it essential for tasks requiring detailed scene understanding and interaction.
How does object detection work?
Object detection typically follows a series of steps:
Image Preprocessing: Enhances image quality and removes noise to facilitate accurate detection.
Feature Extraction: Extracts meaningful features from the image, such as edges, corners, or textures.
Classification: Uses machine learning algorithms to classify each region of interest (ROI) as containing an object or not (binary classification).
Localization: Determines the precise location (bounding box coordinates) of detected objects within the image.
Popular algorithms like YOLO (You Only Look Once), SSD (Single Shot MultiBox Detector), and Faster R-CNN (Region-based Convolutional Neural Network) employ variations of these steps to achieve real-time performance and high accuracy.
What are the main applications of object detection technology?
Object detection technology finds diverse applications across various sectors:
Autonomous Vehicles: Enables vehicles to detect pedestrians, cyclists, and other vehicles for safe navigation.
Healthcare: Facilitates medical imaging analysis for diagnosing diseases from X-rays, MRIs, and CT scans.
Security: Enhances surveillance systems by detecting unauthorized intrusions or suspicious activities.
Retail: Automates inventory management and offers personalized shopping experiences through facial recognition and customer behavior analysis.
What are the benefits of using object detection technology?
Implementing object detection technology offers several advantages:
Enhanced Safety and Security: Real-time monitoring and threat detection in public spaces and critical infrastructure.
Automation and Efficiency: Streamlines processes in manufacturing, logistics, and retail, reducing operational costs and human errors.
Improved User Experience: Personalizes services based on customer preferences and behavior, enhancing satisfaction and loyalty.
Accessibility: Assists visually impaired individuals through object recognition apps and devices, promoting inclusivity in technology use.
What are the limitations of object detection technology?
Despite its advantages, object detection technology faces several challenges:
Detection Accuracy: Difficulty in identifying occluded or overlapping objects, affecting reliability in complex environments.
Environmental Factors: Performance degradation under poor lighting conditions, adverse weather, or ambiguous visual cues.
Complex Implementation: High costs associated with hardware requirements, data storage, and continuous model training and updates.
Regulatory Compliance: Compliance with data protection laws and ethical guidelines regarding privacy and data usage, particularly in surveillance and facial recognition technologies.
What are the ethical considerations surrounding object detection technology?
Object detection technology raises ethical concerns related to:
Privacy: Collection and use of personal data for surveillance purposes, raising concerns about individual rights and data protection.
Bias: Algorithms may exhibit biases based on training data, leading to unfair outcomes in automated decision-making processes such as hiring or law enforcement.
Transparency and Accountability: Ensuring transparency in how object detection systems operate and making developers accountable for the consequences of their technologies.
Regulation: Navigating regulatory frameworks like GDPR (General Data Protection Regulation) in Europe, which sets guidelines for handling personal data and ensuring ethical AI deployment.
How can object detection technology be improved in the future?
Ongoing research and development efforts aim to enhance object detection technology by:
Advancing Deep Learning Techniques: Innovations in neural network architectures and training methodologies to improve accuracy and efficiency.
Integration with AI: Combining object detection with natural language processing and other AI technologies for more comprehensive understanding and decision-making capabilities.
Addressing Technical Challenges: Resolving issues related to object occlusion, lighting variations, and scalability in real-world applications.
Ethical Frameworks: Developing ethical guidelines and frameworks for responsible AI deployment, ensuring fairness, transparency, and accountability in object detection systems.
What are some real-world examples of object detection technology in action?
Real-world deployments of object detection technology include:
Autonomous Vehicles: Companies like Tesla use object detection to enable vehicles to detect and respond to surrounding vehicles, pedestrians, and traffic signals.
Healthcare: Medical imaging analysis tools use object detection to identify and classify anomalies in X-rays and MRIs, aiding in early disease diagnosis.
Retail and Security: Retailers utilize object detection for inventory management and personalized customer experiences, while security firms employ it for surveillance and threat detection in public spaces.
Conclusion
Object detection technology continues to evolve, driving advancements across industries and reshaping how machines perceive and interact with their surroundings. By addressing common questions and concerns, this section aims to provide a comprehensive understanding of the capabilities, challenges, and ethical considerations associated with object detection technology. Looking ahead, continued research and ethical frameworks will play pivotal roles in harnessing the full potential of object detection while ensuring responsible and beneficial deployment in society.
This expanded FAQs section provides detailed insights into various aspects of object detection technology, offering readers a comprehensive overview and understanding of its implications and applications in modern society.
Disclaimer and Caution: Object Detection Technology
Introduction
Object detection technology has rapidly advanced, offering numerous benefits across various industries. However, like any technology, it comes with inherent risks and considerations that users and developers must be aware of to ensure responsible deployment and use.
Understanding Object Detection Technology
Object detection technology utilizes computer vision algorithms to identify and locate specific objects within digital images or video frames. It plays a crucial role in applications ranging from autonomous vehicles and healthcare to security and retail. By enabling machines to perceive and interact with their environments, object detection enhances automation, efficiency, and safety in numerous sectors.
Disclaimer: Limitations and Considerations
While object detection technology offers significant advantages, it’s important to acknowledge its limitations and potential risks:
Accuracy and Reliability:
Object detection systems may not always accurately identify objects, especially in challenging conditions such as low light or cluttered environments. Users should understand the technology’s capabilities and limitations before relying on it for critical tasks.
Environmental Factors:
Performance can vary based on environmental factors like weather conditions, object occlusion, and camera angles. Developers should conduct thorough testing across diverse scenarios to ensure robust performance in real-world applications.
Data Privacy and Security:
Implementing object detection often involves collecting and processing sensitive data, including images and videos. It’s essential to adhere to data protection laws and ethical guidelines to safeguard user privacy and prevent misuse of personal information.
Ethical Considerations:
Object detection systems may inadvertently perpetuate biases present in training data, leading to unfair outcomes in decision-making processes. Developers must prioritize fairness, transparency, and accountability to mitigate ethical concerns and promote inclusive technology deployment.
Caution: Implementation and Deployment
Effective and responsible implementation of object detection technology requires careful planning and consideration of various factors:
Clear Objectives and Use Cases:
Define clear objectives and use cases for implementing object detection technology. Understanding specific requirements helps in selecting appropriate algorithms, hardware, and data processing techniques.
Compliance with Regulations:
Ensure compliance with relevant regulations and standards governing data privacy, security, and ethical AI deployment. Familiarize yourself with frameworks like GDPR in Europe or CCPA in California to avoid legal implications.
Risk Assessment and Mitigation:
Conduct thorough risk assessments to identify potential vulnerabilities and risks associated with object detection systems. Implement mitigation strategies to address identified risks and ensure system resilience.
Testing and Validation:
Rigorous testing and validation are crucial to verify the accuracy, reliability, and performance of object detection models across different scenarios and conditions. Consider using diverse datasets and conducting field trials to assess real-world performance.
User Education and Training:
Provide adequate training and education to users and stakeholders involved in deploying and using object detection technology. Foster awareness of its capabilities, limitations, and ethical considerations to promote responsible usage.
Best Practices for Responsible Deployment
To maximize the benefits of object detection technology while minimizing risks, adhere to best practices:
Continuous Monitoring and Maintenance: Regularly monitor system performance and conduct maintenance to ensure optimal functionality and reliability.
Transparency and Accountability: Maintain transparency in how object detection systems operate and ensure accountability for decisions made based on system outputs.
Feedback and Improvement: Solicit feedback from users and stakeholders to identify areas for improvement and refine object detection models over time.
Collaboration and Engagement: Foster collaboration with industry peers, researchers, and regulatory bodies to stay informed about emerging trends, best practices, and regulatory updates.
Conclusion
Object detection technology holds immense promise in transforming industries and improving societal outcomes. However, its responsible deployment requires careful consideration of technical capabilities, ethical implications, and regulatory requirements. By understanding the limitations, addressing potential risks, and adhering to best practices, stakeholders can harness the full potential of object detection technology while ensuring ethical integrity and user trust.
This disclaimer and caution section provides a comprehensive overview of the considerations and best practices necessary for the responsible deployment and use of object detection technology. It emphasizes the importance of understanding limitations, addressing risks, and fostering transparency and accountability in its implementation.