Data Privacy Challenges in AI: Strategies and Best Practices
Introduction
As artificial intelligence (AI) continues to permeate various sectors, concerns about data privacy have become more pronounced. This article explores the key challenges businesses face in ensuring data privacy within AI systems and provides actionable strategies and best practices to mitigate these risks.
Success Stories and Exemplary Cases
Key Challenges in Data Privacy for AI
Table 1: Challenges in Data Privacy for AI
Challenge | Description |
---|---|
Lack of Transparent Data Handling | AI systems often process large volumes of sensitive data without clear user consent mechanisms. |
Algorithmic Bias and Fairness | Biased AI algorithms can perpetuate discriminatory practices, raising ethical and legal concerns. |
Security Vulnerabilities | Weaknesses in AI systems’ security measures can lead to data breaches and unauthorized access. |
Strategies and Best Practices
Table 2: Strategies for Ensuring Data Privacy in AI
Strategy | Description |
---|---|
Data Minimization | Collect and store only necessary data for AI training and operations. |
Privacy by Design | Integrate privacy features into AI systems from the outset of development. |
Regular Audits and Assessments | Conduct periodic reviews to ensure compliance with evolving privacy regulations. |
Conclusion
In conclusion, safeguarding data privacy in AI requires a proactive approach that balances innovation with ethical considerations. By implementing robust strategies and best practices, businesses can foster trust among users and mitigate potential risks associated with AI-driven data processing
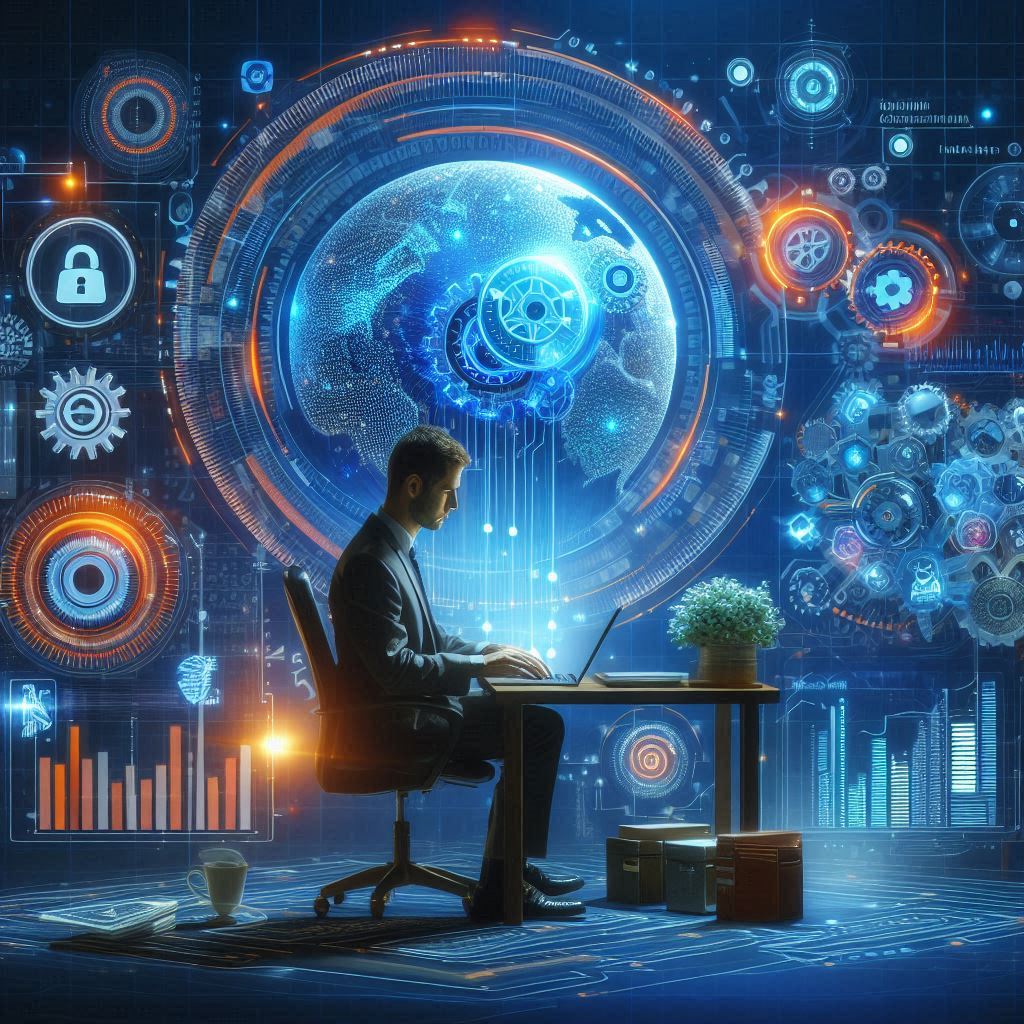
Introduction
Brief introduction to the growing influence of AI across industries.
Importance of understanding both advantages and challenges for effective deployment.
Pros of AI
Enhanced Efficiency
Examples of AI streamlining processes in industries like manufacturing, healthcare, and finance.
Statistical data showing improved productivity and reduced operational costs.
Data Analysis and Insights
AI’s ability to process vast amounts of data quickly and accurately.
Applications in market analysis, customer behavior prediction, and decision-making.
Automation of Repetitive Tasks
How AI automates mundane tasks, freeing up human resources for more complex activities.
Real-world examples of automation leading to increased productivity and job satisfaction.
Personalization and Customer Experience
AI’s role in providing personalized recommendations and customer service.
Case studies from e-commerce and entertainment sectors showcasing enhanced user experiences.
Innovation and Creativity
AI’s contribution to creative fields like art, music, and literature.
Discussion on AI-driven innovations that wouldn’t be possible without machine learning algorithms.
Cons of AI
Job Displacement and Economic Impact
Concerns about AI replacing human jobs in various sectors.
Studies and statistics on job displacement and economic repercussions.
Ethical and Social Implications
Issues surrounding AI ethics, including bias in algorithms and data privacy concerns.
Examples of controversial AI applications and their societal impacts.
Dependency on Technology
Risks associated with over-reliance on AI systems without adequate human oversight.
Instances of system failures and their consequences in critical sectors.
Security and Privacy Risks
Threats posed by AI-powered cyberattacks and data breaches.
Examples of security vulnerabilities and their implications for businesses and individuals.
Regulatory Challenges
Challenges in regulating AI technologies to ensure ethical use and accountability.
Comparison of international approaches to AI regulation and their effectiveness.
Conclusion
Summary of the dual nature of AI’s impact, highlighting the need for balanced deployment.
Future outlook on addressing challenges and maximizing benefits through responsible AI development.
This outline provides a structured approach to discussing both the positive and negative aspects of AI, allowing for in-depth exploration and analysis within a 1000-word article.
[…] the digital age, data privacy has become a paramount concern for individuals and organizations alike. As data breaches and cyber […]